Anomaly detection in satellite telemetry is critical in its safe operation and is one of the prioritized use cases in the ESA Artificial Intelligence for Automation (A2I) Roadmap. Anomaly detection is an active area of research in which multiple classic and machine learning algorithms were proposed to identify potential anomalies in time series [1]. However, satellite telemetry is a special case of time series characterized by high dimensionality (thousands of parameters per satellite), missing data (idle states and varying sampling rates during a mission), and – most importantly – limited information about known anomalies. These were the main problems identified during our “On-board anomaly detection from the OPS-SAT telemetry using deep learning” [2] where just a few expert-annotated anomalies were available. It is a common situation in many missions because preparing a comprehensive curated list of anomalies is a time-consuming and user-dependent task that can be treated as a separate project itself (such as ESA Anomalies Dataset For International AI Anomaly Detection Benchmark T709-705OS project which we are involved in) and sometimes there are no more than a few anomalies present in the telemetry, especially in the beginning of the mission. Moreover, most of the existing (deep and shallow) anomaly detection methods are not able to leverage prior knowledge (e.g., a few labeled anomalies) when such information is available. This problem can be addressed with a few-shot learning approach popularized in the domain of computer vision (e.g., MSMatch algorithm for multispectral scene classification developed by ESA Advanced Concepts Team [3]) but, to the best of our knowledge, this approach has never been applied to satellite telemetry anomaly detection.
_______
[1] A. Blázquez-García, A. Conde, U. Mori, and J. A. Lozano, “A Review on Outlier/Anomaly Detection in Time Series Data,” ACM Comput. Surv., vol. 54, no. 3, p. 56:1-56:33, Kwiecie 2021, doi: 10.1145/3444690.
[2] J. Nalepa et al., “Toward On-Board Detection Of Anomalous Events From Ops-Sat Telemetry Using Deep Learning,” presented at the 8th International Workshop On On-Board Payload Data Compression, Athens, 2022, p. 7. doi: 10.5281/zenodo.724499.
[3] P. Gómez and G. Meoni, “MSMatch: Semisupervised Multispectral Scene Classification With Few Labels,” IEEE Journal of Selected Topics in Applied Earth Observations and Remote Sensing, vol. 14, pp. 11643–11654, 2021, doi: 10.1109/JSTARS.2021.3126082.
[4] G. Pang, L. Cao, L. Chen, and H. Liu, “Learning Representations of Ultrahigh-dimensional Data for Random Distance-based Outlier Detection,” in Proceedings of the 24th ACM SIGKDD International Conference on Knowledge Discovery & Data Mining, New York, NY, USA, Lipiec 2018, pp. 2041–2050. doi: 10.1145/3219819.3220042.
[5] Y. Koizumi, S. Murata, N. Harada, S. Saito, and H. Uematsu, “SNIPER: Few-shot Learning for Anomaly Detection to Minimize False-negative Rate with Ensured True-positive Rate,” in ICASSP 2019 - 2019 IEEE International Conference on Acoustics, Speech and Signal Processing (ICASSP), May 2019, pp. 915–919. doi: 10.1109/ICASSP.2019.8683667.
[6] G. Pang, C. Shen, and A. van den Hengel, “Deep Anomaly Detection with Deviation Networks,” in Proceedings of the 25th ACM SIGKDD International Conference on Knowledge Discovery & Data Mining, New York, NY, USA, Lipiec 2019, pp. 353–362. doi: 10.1145/3292500.3330871.
[7] W. Zhang and C. Challis, “Weakly Supervised Anomaly Detection for Streaming Data,” in 2021 IEEE International Symposium on Multimedia (ISM), Nov. 2021, pp. 31–34. doi: 10.1109/ISM52913.2021.00013.
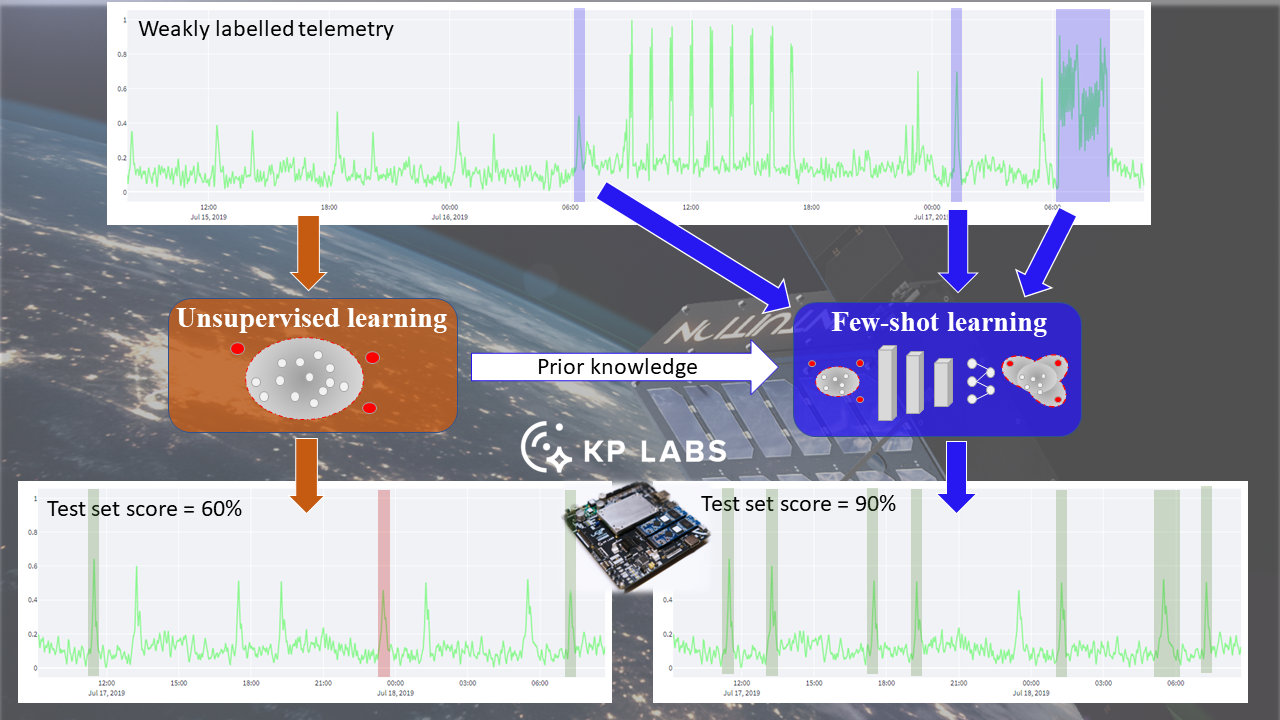