Detecting anomalies from the satellite telemetry is critical in its safe operation. Classical solutions for this task often utilize the out-of-limit checks, which allow us to find point anomalies. To detect other types of events, such as contextual or collective ones, we can exploit data-driven deep learning-powered models – this project will build upon the current advances in the field, and we will incorporate recurrent neural networks into the proposed event detection pipeline that will be deployed on-board OPS-SAT. Additionally, we will explore the possibility of detecting anomalies from multi-modal sequential signals, e.g., captured by different components of the satellite, as such telemetries may highlight the events that would not be possible to detect using a single channel exclusively. Overall, our main objective is to demonstrate that deep learning can be effectively deployed on-board OPS-SAT for automated event detection from its operational telemetry data. We hope that the project will be an important step towards data-driven Fault Detection, Isolation and Restoration systems that will ultimately improve the safety of emerging satellites, and reduce their operational costs through delivering clear insights into the behavior of the entire spacecraft and its pivotal (sub)systems.
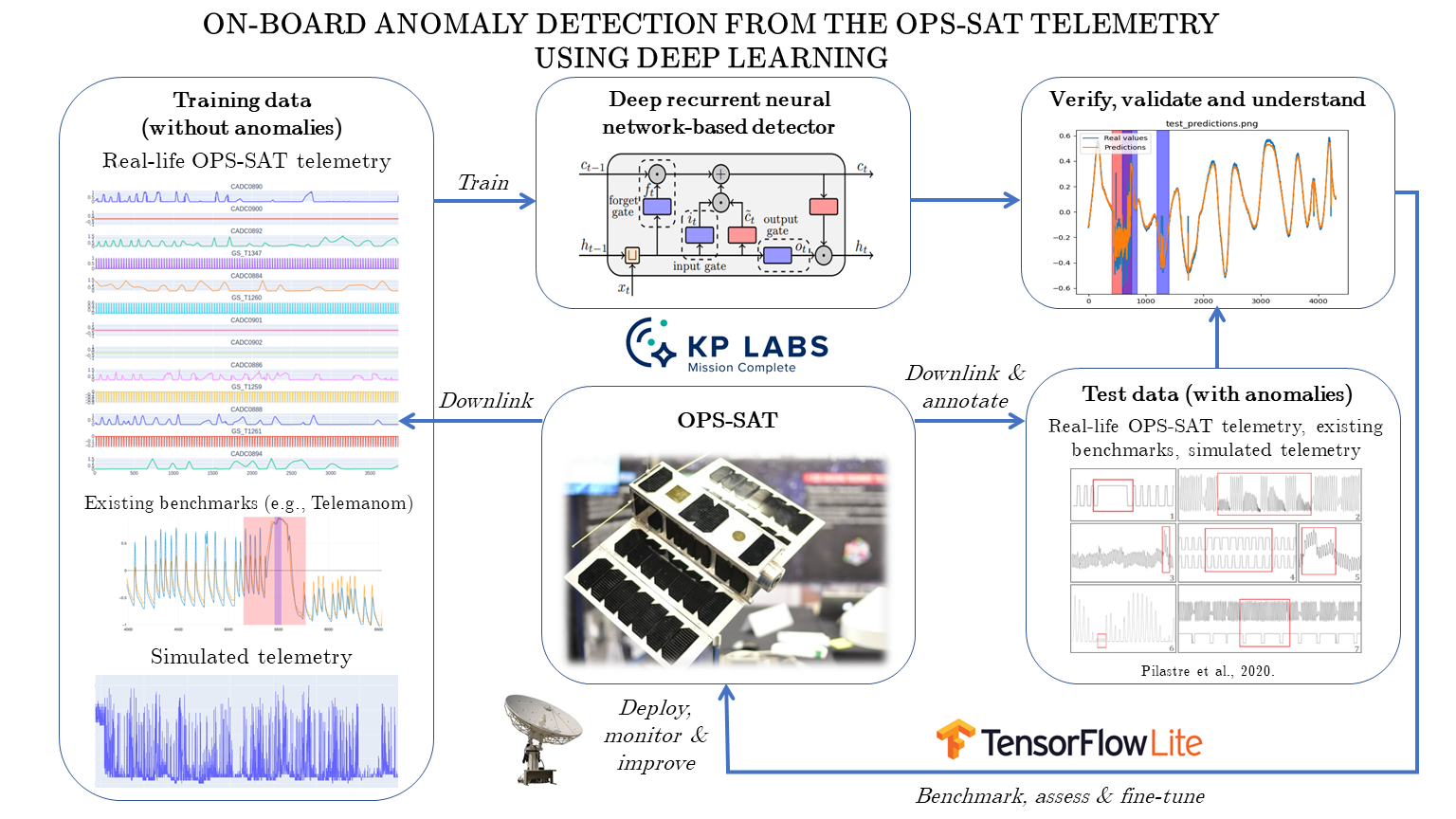