Cyberattacks on aerospace systems are becoming a growing concern for space missions, although they are often unpublished in order to attempt to delay or avoid further hacking. Cyberattacks in space pose serious risks for ground based critical infrastructure, and insecurities in the space environment. Thus, an important consideration to preserving future critical space mission objectives is a successful detection and appropriate defensive response to an adversarial attack on AI based space software solutions. Explainable Artificial Intelligence (XAI) techniques are becoming a powerful method for space missions to sensibly acting in explaining the AI techniques outcomes especially when dealing with unanticipated and complex situations. We, therefore, harness the XAI techniques with adversarial learning for adversarial attacks detection, specifically on the embedded spacecraft Guidance Navigation and Control (GNC) based AI systems. The XAI algorithms will be developed to make onboard adversarial learning transparent to ensure a trustable decision, providing highly precise detection and defensive response to ensure the space vehicle safety. A comprehensive framework will be studied and proposed to address the XAI-based adversarial learning for spacecraft GNC systems, including synthetic datasets/real data generation, guidance scenario building, XAI-based adversarial learning model development, software testing, etc. In our work, we first develop an XAI based deep learning model providing the performance required for the GNC scenario proposed. Then an XAI adversarial learning method that handles the challenging detection through classification of adversarial attacks and input distribution shifts with a good explanation of results is proposed. We also focus on improving the network robustness and propose and extension to adversarial training and finally we conduct extensive validation of the proposed architecture based on simulation and real data .
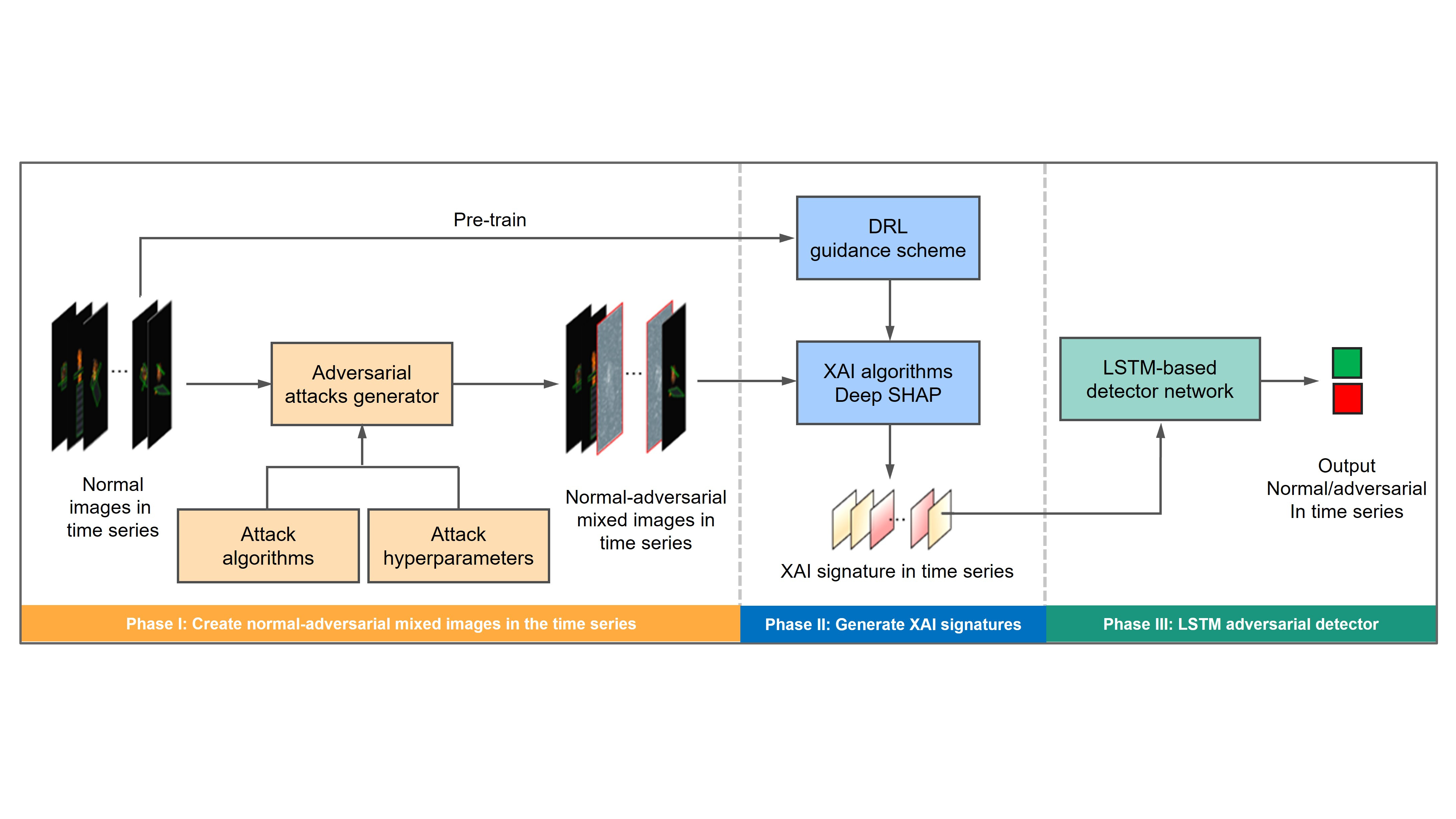