The project aims at the development of a novel system based on Artificial Intelligence (AI) in which an agent is taught to control the OPS-SAT and actively track Earth features (targets). It is named Deep Active Tracking (DAT) system. Two AI-based algorithms are placed at the core of the system.
The first one is a Computer Vision (CV) algorithm that processes the information from images taken by the OPS-SAT HD optical camera to detect the selected targets. Their location is passed in the second algorithm. This is a smart agent that decides the optimal sequence of actions to place and keep the target centred in the images. As a result, the DAT system controls the attitude of the OPS-SAT and tracks the targets by directly acting on its reaction wheels, only using the images provided by the OPS-SAT optical camera as input.
Both the computer vision and the smart agent algorithms are built on neural networks that are specifically trained to master in their tasks. CV algorithm is trained using supervised Deep Learning technique from a labelled dataset of images of the Earth features selected to track. The smart agent is trained by means of Reinforcement Learning and interaction with a simulated environment of the OPS-SAT dynamics and target kinematics.
Once developed and trained, the DAT system is to be deployed in the OPS-SAT and connected to its optical camera and reaction wheels. Then the system will be ready for testing in-orbit where it will undergo an exhaustive test plan to verify its performance and judge the generalization capacity of the smart agent to deal with non-trained situations.
The end of the project seeks to prove the feasibility of the DAT system. A successful outcome will pave the way to upgrade the tracking and observation capacities of other satellites, with minor adaptation to the DAT system. It will also show the potential of a new generation of systems based on AI that are able to get more from current non-smart COTS elements at a low cost.
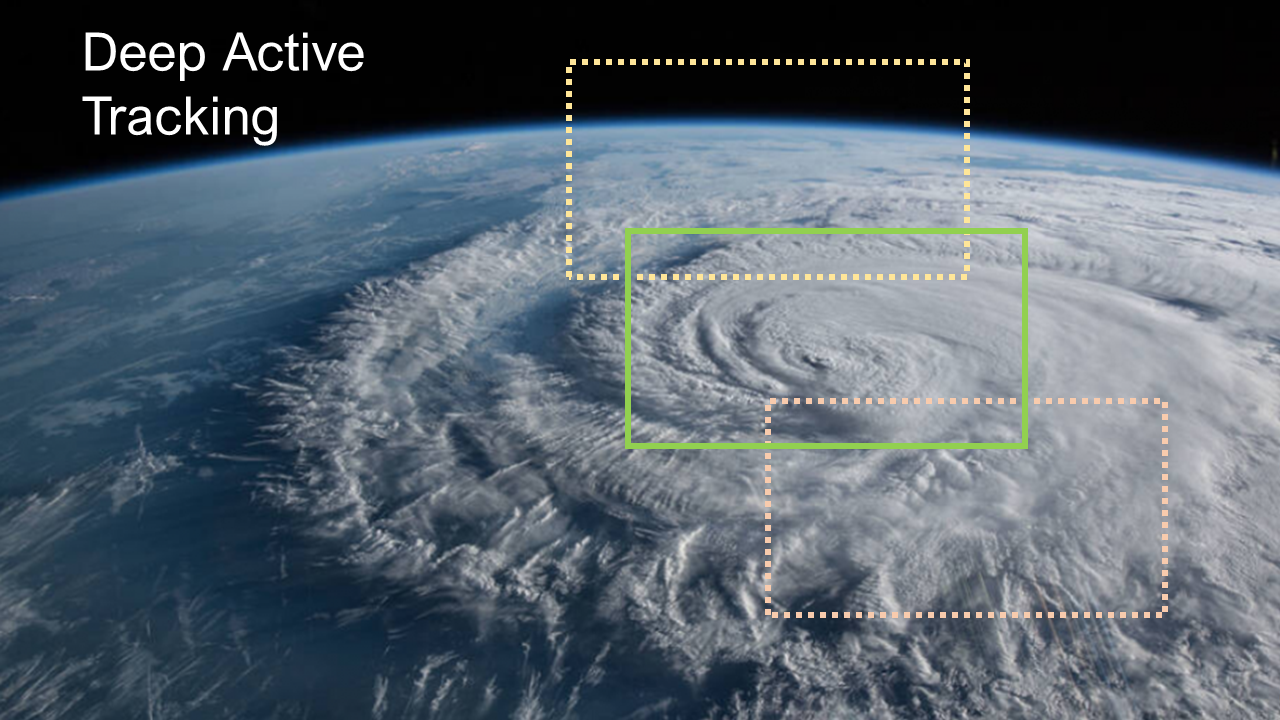