
Running
The project aims at training deep learning convolutional neural networks to characterise astronomical objects based on all their multi-wavelength pixel distributions at all images from ESA observatories that have seen that object and then could support users in exploring the contents of the data archives, not based on the image metadata, as it is currently done in all astronomical archives world-wide, but based on the semantic contents of the data itself. This research project will set the basis to enable future data-driven and pattern-driven searches on these vast ESA Astronomical data archives where the users can ask about the occurrence of physical objects of certain characteristics such as e.g. high-proper motion young binary stars with circumstellar disks or unpublished high-redshift galaxies with hard X-ray counterparts. These type of searches will allow the analysis of astronomical archival data to enter into the XXI Century by tapping on the spectacular progress in image recognition from the AI industry from the last years and will prepare ESA scientists and the scientific community using ESA data to deal with the avalanche of high-quality data from the Gaia and Euclid upcoming data releases. We will combine the extensive Machine Learning expertise at the Centre for Doctoral Training on Data Intensive Science from the University College London with our expertise on ESA data at ESA's Science and Operations department.
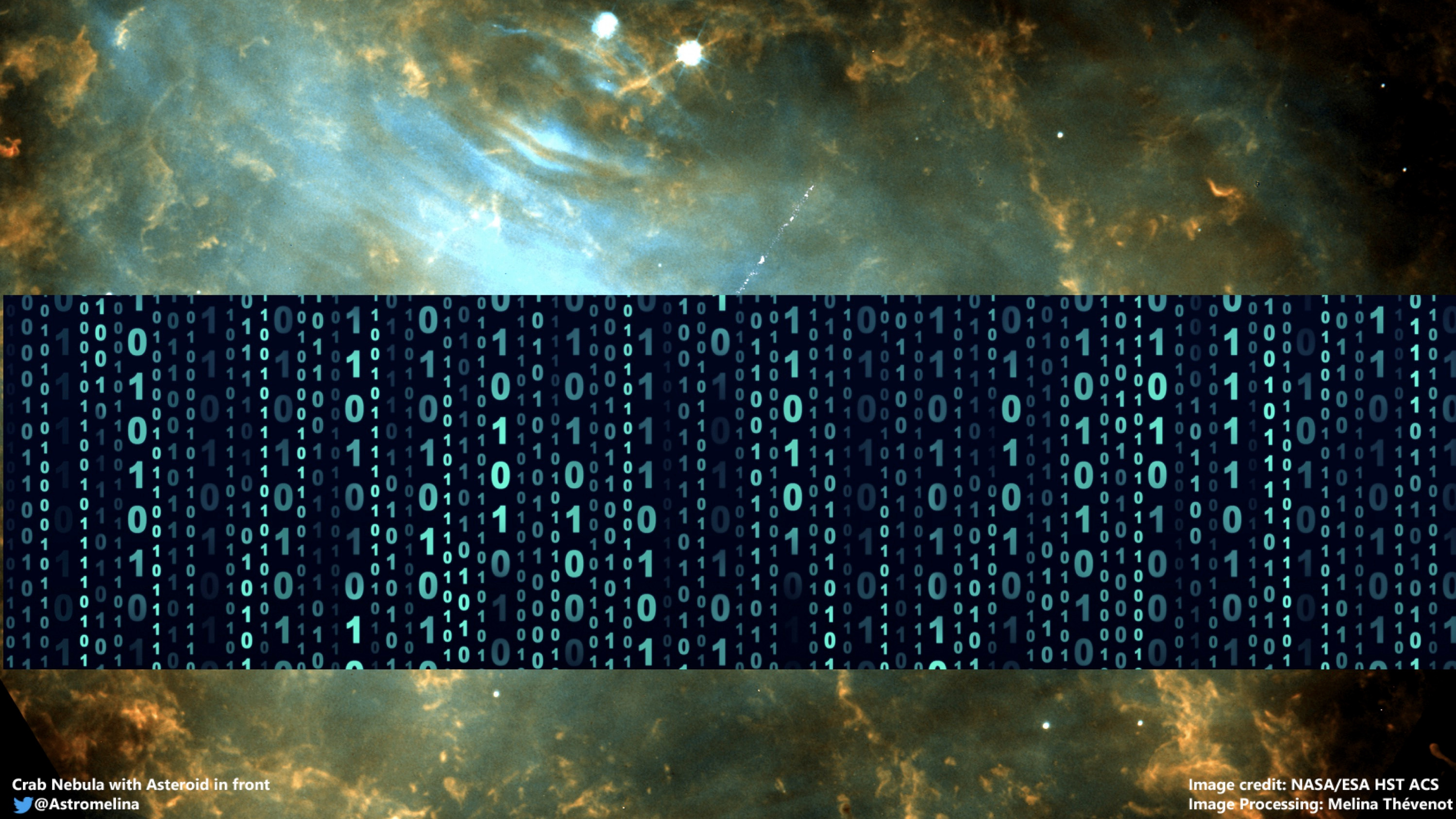