Duration: 36 months
The recent successes of AI-based capabilities have created new opportunities in space exploration. In 2020, the first mission using Deep Learning AI Earth observation algorithms onboard Φ-Sat-1 was launched [1]. From that point, several hardware architectures have enabled AI onboard satellites: soft GPU, COTS processors, and systolic-arrays architectures [2 - 8]. Among the various European solutions, the most advanced technology is based on the 28nm FDSOI technology. However, this technology has certain limitations in terms of performance when compared to US competitors, such as the AMD VERSAL, which uses the 7nm FinFET technology node [9]. The proposed project aims at overcoming flexibility and energy-efficiency current limitations, by paving the way to implementing an AI Engine IP core on ultra-deep sub-micron (UDSM) technology. The UDSM is a disruptive high-performance technology upcoming in the space industry and capable of increasing the energy efficiency of space components [10]. As an AI hardware accelerator core, we leverage a resource-optimized, energy-efficient Coarse-Grained Reconfigurable Array (CGRA) [11] [12] alongside a RISC-V-based SmartDMA, orchestrating the memory transactions. Algorithm execution will comprise the deployment of the scheduling algorithm on the RISC-V CPU and the configuration of the CGRA processing core. The project will deal with the system integration and verification in Hardware Description Language (HDL) of the AI Engine, its implementation and characterization with a 7nm USDM technology employing the radiation hardening by design concept. The AI Engine IP core will be exploitable as an IP/chiplet in complex payload data handling systems and aims to enrich the European library characterisation of USDM technology. This idea aligns with the ESA's Technology Strategy [13], and has already gained interest from the industry (NanoXplore [14]) and support from ESA staff.
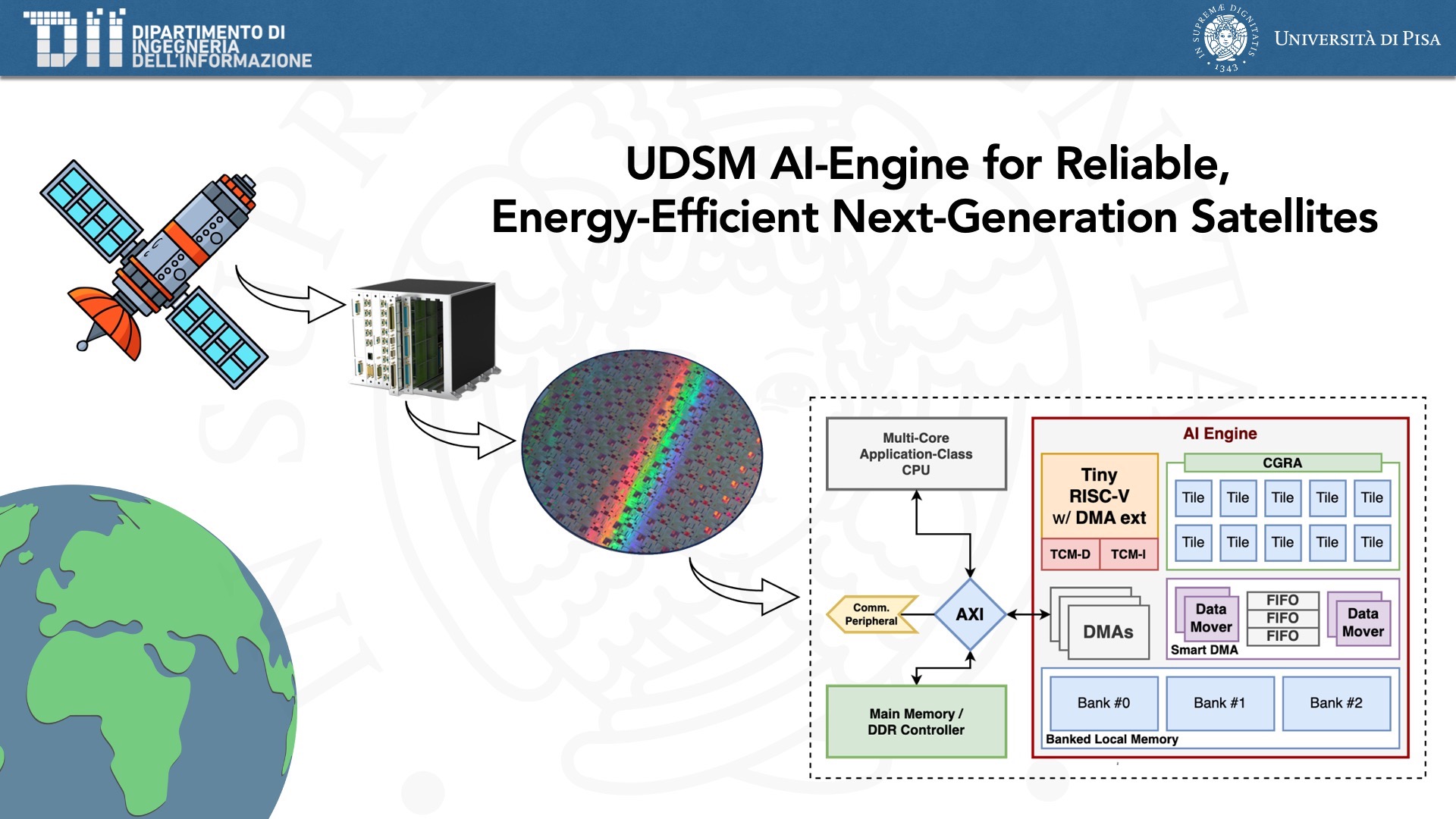