This project aims to build the first working prototype of a language-powered foundation model (FM) for remote sensing (RS). To this end, we will employ self-supervised contrastive learning of multi-sensor Earth observation (EO) data with language semantics. The resulting FM will be adaptive to new tasks, sensor agnostic and rooted in human understandable semantics. FMs have attracted great attention in RS due to their potential to: 1) model massive EO data with self-supervised learning; 2) generalize well to various EO problems; and 3) jointly leverage language and vision reasoning. However, the limitations of the existing precursors of FMs prevent unleashing their full power for large-scale information discovery from EO data archives. First, they are mostly based on pixel-level reconstruction of masked RS images, and thus the resulting representations embody low semantic levels. Second, they utilize RS images acquired by a single sensor but mostly lack multi-sensor vision reasoning capabilities. Third, the existing vision-language models learn image-text correspondences with limited textual sources (e.g., auto-generated sentences from class labels), and thus cannot learn in-depth language semantics specific to EO problems. To build the first FM prototype for EO, multi-sensor RS images acquired on the same area with different timestamps, as well as the corresponding geotagged text data, are considered as the different views of a shared context. Then, modality-specific transformers will be developed, enforcing georeferenced positional encoding strategies to model mutual information between these views. Specialized losses and strategies for missing or incomplete modalities will be researched. In addition, we will exploit a language modeling approach for autoregressive learning of EO-specific language semantics. The resulting FM will be compatible with RS images from different sensors with variable spatial resolutions, missing data and image-grounded language reasoning.
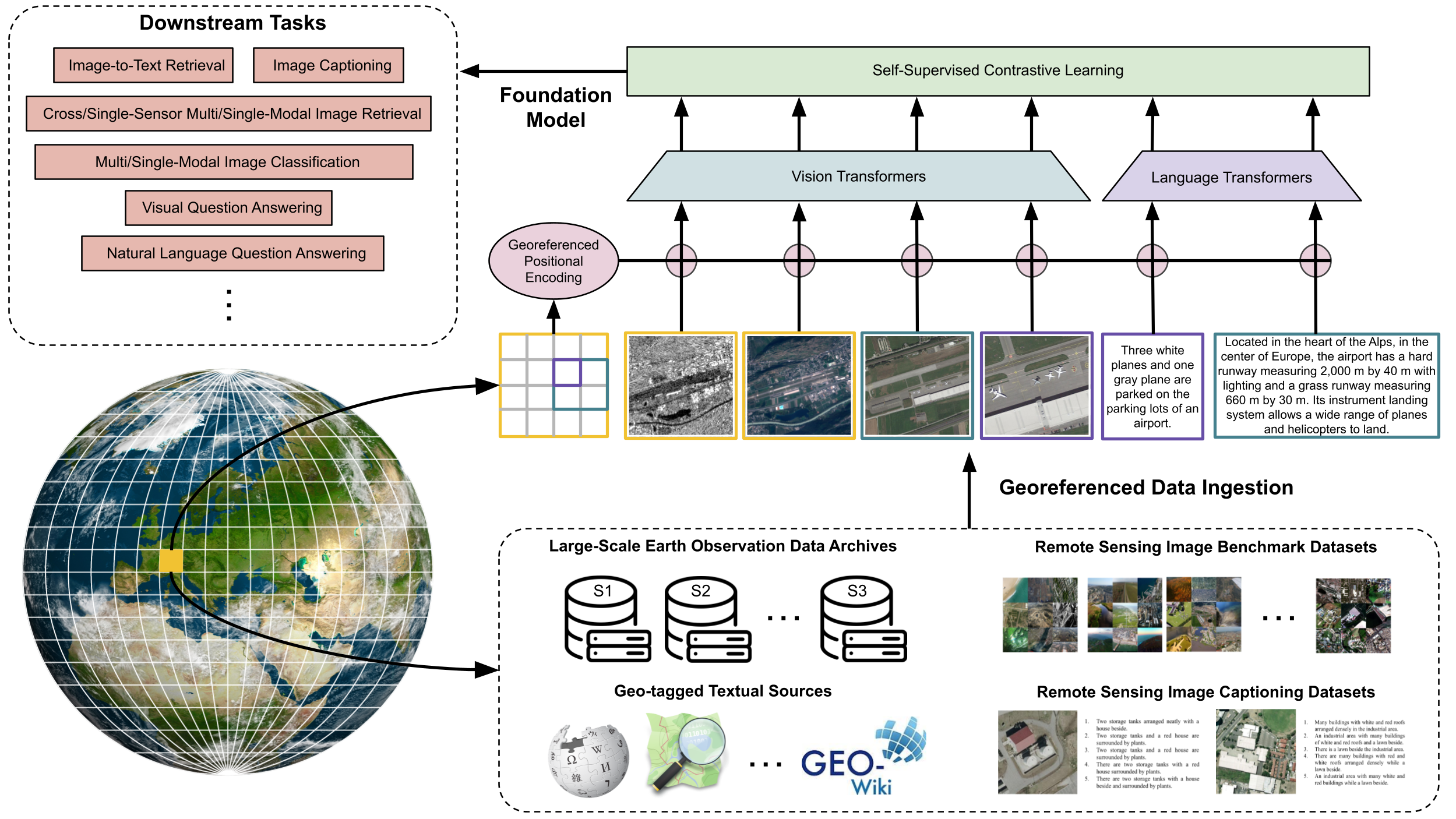