Duration: 42 months
Estimating the asteroids' density accurately is of great importance for both planetary science and defense to unlock our understanding of their formation, improve our knowledge of the gravity environment around them and enhance the safety of spacecraft-asteroid proximity operations. Asteroids are the result of impacts between celestial objects, thus, gravity anomalies in asteroids can occur. Indeed, the Moon is characterised by such anomalies (mascons) which can affect the orbit stability of a spacecraft. We propose a novel approach to explore gravity anomalies in asteroids by reconstructing their density distribution, combining traditional measurements of gravity-induced acceleration, experienced by a spacecraft, with dynamical stability indicators which has yet to be explored. Traditional methods to estimate the asteroids’ internal density distribution include the fitting of gravity coefficients from a chosen gravity model combined with the gravity accelerations experienced by a spacecraft. Several inverse constrained optimisation methods have been explored such as least-squares, Bayesian, neural network, and genetic algorithms. We propose a novel machine learning approach to the inverse problem with constraints from the dynamical systems theory (e.g., inverse problem from equilibrium points detection as shown in the Fig.) which differs from traditional approach that makes use of solely gravity-induced accelerations. We aim at developing a strategy for autonomous radio science operations, thus developing the onboard software that enables a spacecraft or CubeSats to self-estimate the internal grain density distribution of asteroids. This study will enable, for example, a generalised strategy for determining sequences of spacecraft’s close-proximity ascending operations (e.g., hovering, orbit) for estimating asteroids' internal structure as a function of their physical properties (e.g., class, size, spin-ratio).
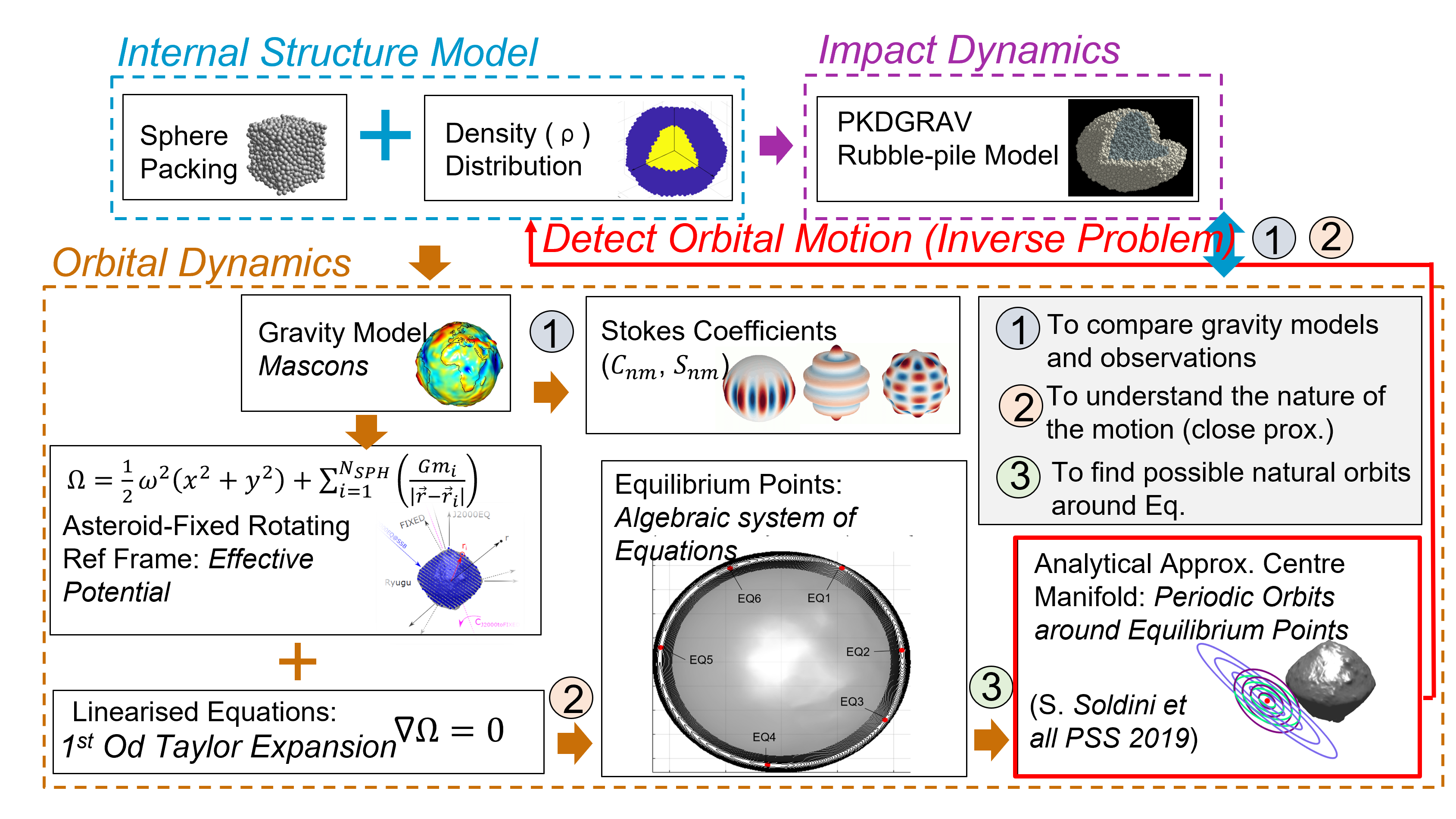