Additive manufacturing (AM) technologies are considered an essential tool since the latest industrial revolution, Industry 4.0. For space applications, it is a key enabling technology, as fairing volume and cargo load limitations are critical drivers in numerous mission scenarios. AM techniques can enable lightweight, in-situ, on-demand manufacturing, thereby producing mass-optimised parts and reducing the amount of spares shipped from Earth. This applies to structural spacecraft parts, but also to future in-situ bioprinting, to support long term crewed missions. The first step required in AM, is the development of a 3D model of the part. Classic CAD design involves a time-consuming loop, where this model is created manually, tested, and then corrected for the next analysis iteration. In addition, 3D slicer programs used in AM, usually allow only regular lattice patterns for the internal architecture of parts (infill), which facilitates crack propagation and failure of structures, in particular when manufacturing defects are present. Regular patterns are also not representative of most biological structures. Our aim within this activity is to develop a set of algorithms that allow us to create more robust graph-based organic structures in an autonomous way, given a basic set of initial parameters. Henceforth we aim to use physics-informed neural networks (PINNs) which will not require initial big data, as internal relations replicate physical laws. It will be an iterative computational design model, representing a cyclic data flow pipeline. The outcome of this project will help to obtain better infills for 3D objects that avoid crack propagation and fit the desired physical environment (in regard to internal flow and external forces). The final application of such structures may be a biological scaffold for tissue engineering or any other lightweight porous structure (especially for space: structural parts for spacecraft, habitats or other infrastructure).
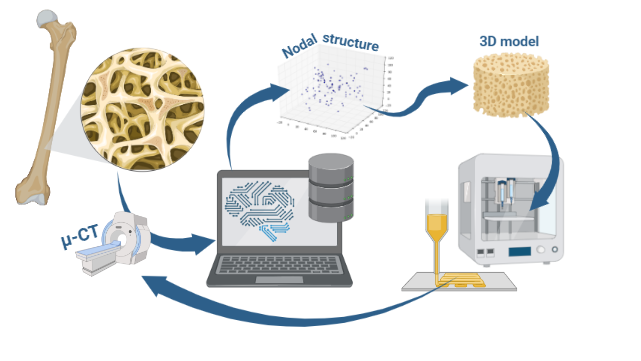