
Running
Characterising the atmospheres of extrasolar planets -planets orbiting other stars- allows us to understand these alien worlds' chemistry, thermal environment, and formation histories. Complex chemical modelling of exoplanetary atmospheres is an essential stepping stone toward detecting biosignatures in one of the nearby exoplanets in the future. With the imminent launch of the James Webb Space Telescope and the launch of the ESA Ariel mission in 2029, we are entering a new era of galactic planetary science. This new wealth of data and its high quality poses unprecedented challenges in modelling the complex behaviour of these exotic atmospheres. Current atmospheric modelling techniques are severely limited in their complexity by computational run-time constraints. The current generation of oversimplified models is insufficient to explain future JWST and Ariel data accurately. Here, recent developments in Artificial Intelligence (AI) offer novel solutions to traditionally insurmountable barriers. In collaboration with Bruno Merín and the machine learning group at the ESAC Science Data Centre, we plan to address the current bottlenecks by leveraging recent advances in graph neural networks and ExplainableAI. These will allow us to develop deep learning solutions that more efficiently model complex interdependencies in data. Successfully modelling the correlations in complex chemical pathways requires an understanding of clustering solutions and cluster interdependencies. This is conceptually similar to classifying astronomical object clusters in extensive data archives. Hence this work finds direct applications to fast data-driven searches of ESA archives by developing next-generation sampling/optimisation techniques.
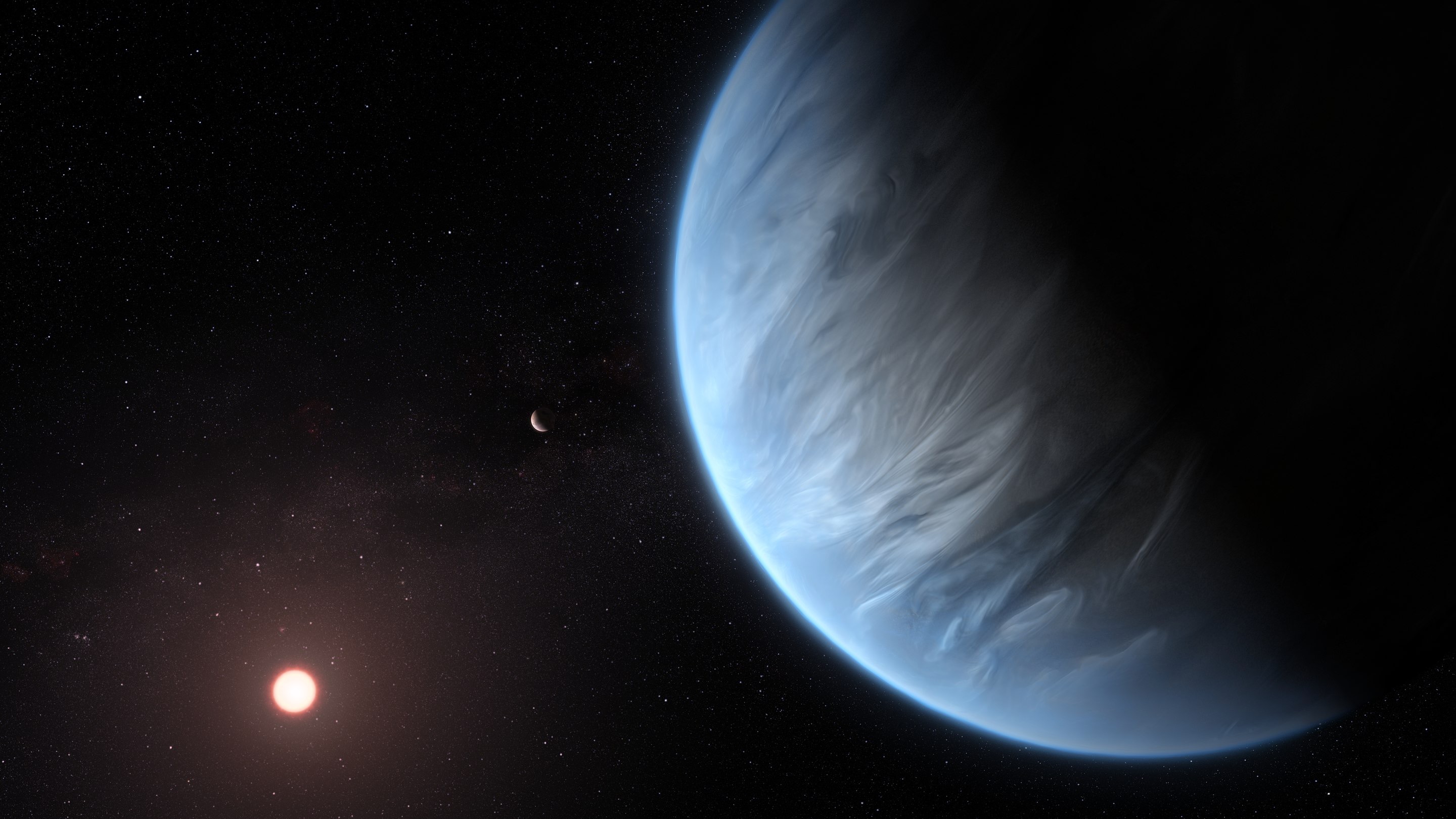