Project HyperClass (Hyperspectral space object Classification) aims at demonstrating the use of hyperspectral and multispectral analysis of the light absorbed and reflected by space objects to acquire a knowledge of their surface composition, better reconstruct their attitude motion and classify them. The key idea is to decompose the light coming from space objects into individual wavelengths and monitor the change in intensity overtime at each wavelength. Since different materials will absorb and reflect differently, by measuring the intensity at different wavelengths one can identify the composition of the surface of a space object and by monitoring the variation of this intensity overtime one can partially reconstruct the attitude motion. Project HyperClass will build a simulation model of the variation of the intensity per wavelength and will use a classifier to associate a lightcurve to a type of object. Then we will experiment with the use of Generative Adversarial Network to relate lightcurve readings to a model of attitude motion for different types of space objects with typical surface compositions. Since space objects have irregular surfaces with different components, from solar arrays to thermal blankets, we will simulate different configurations with different surface elements. Two situations will be simulated: one in which the sensor is in space and is observing objects in Low Earth Orbit from an orbit lower than 500km; the other in which the sensor is on the ground connected to an optical telescope. The simulation model will then be calibrated against laboratory experiments where we will perform a spectral analysis of the light coming from a small satellite, like a CubeSat, for different attitude configurations.
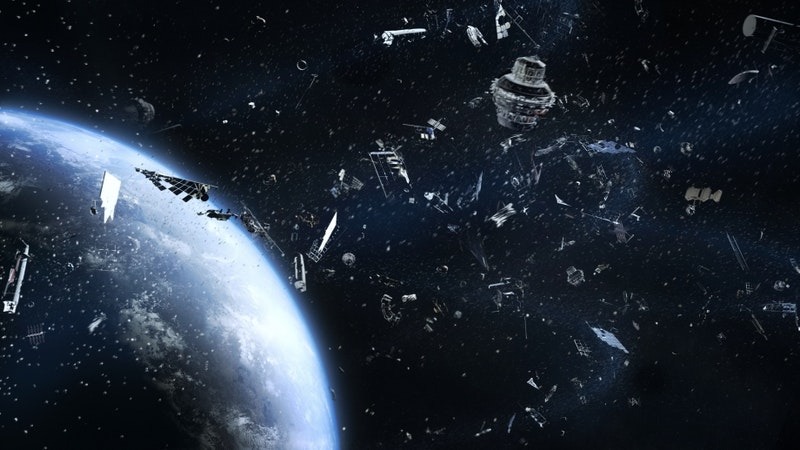