There are two sets of modelling paradigms for estimating environmental parameters from Earth Observations (EO). On the one hand, there exists a group of theory-driven physical models for various processes (e.g., Radiative Transfer Models). Although these models are highly interpretable, they are often imprecise. Data-driven models acquired from machine learning algorithms, on the other hand, offer substantial benefits in improving precision by capturing patterns in EO data. These, however, require large amounts of labelled instances and may lead to physically implausible results. The complementary nature of these modelling paradigms encourages exploring their combination in hybrid modelling. However, configuring and calibrating hybrid models for users in charge of modelling are extremely difficult tasks that require expertise in different fields (familiarity with physical and machine learning models). This creates barriers and challenges regarding the accessibility of these models to machine learning experts and to EO scientists. Our proposed research aims at addressing such problems by exploiting and building upon the advancements in the area of neural architecture search (NAS), a sub-field of automated machine learning (AutoML). The goal of this growing field is to make machine learning available to non-machine learning experts by generating high-performance machine learning pipelines or neural network architectures in a fully automated manner. Our novel idea in this research is to augment the models produced by NAS AutoML systems with the knowledge acquired from physical models by automatically generating neural network architectures that allow creating “an ensemble of models” of different nature (i.e., various interacting physical models, data-driven models). To perform this research, we build upon our ongoing collaborations with ESA-Phi-Lab.
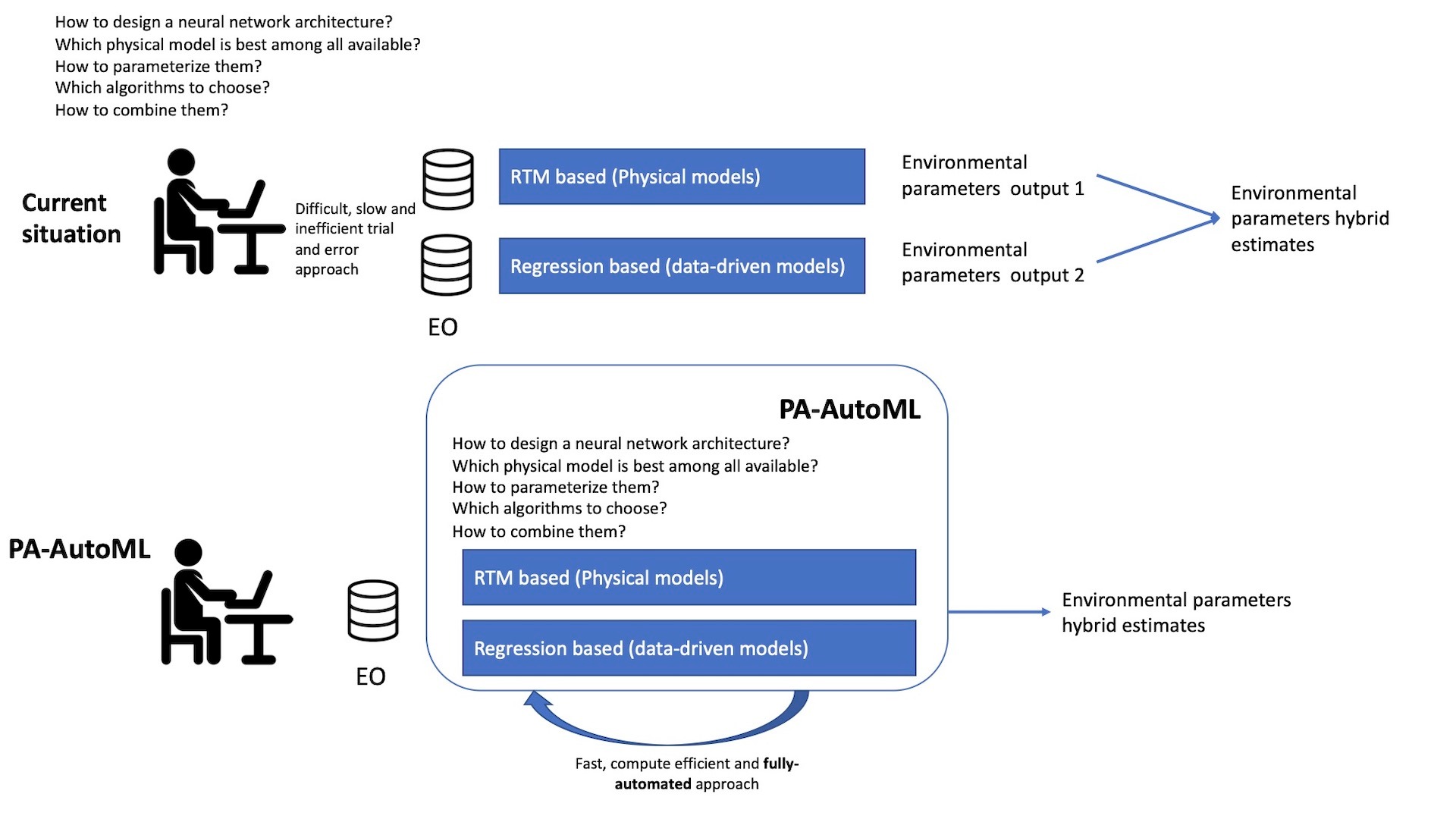