The observational uncertainties of asteroids, combined with the chaotic nature of the solar system, makes predicting their future positions an inherently difficult task, as small deviations in the assumed orbits of the objects can lead to extraordinary variation in their final positions. Conventional Monte-Carlo approaches attempt to remedy this problem by running thousands, or sometimes millions, of simulations based off of an assumed parameter distribution. This approach, however, may still not sufficiently explore all the possible outcomes. Building off of the concepts presented in our recent publication [J.D. Hefele, F. Bortolussi, S.F. Portegies Zwart (2020)], we would like to take the next steps in creating an AI enhanced Earth impact monitoring system that initially identifies hazardous objects based off of their orbital topologies. The system would first select "potential impactors" using an neural network that was trained on the orbits of simulated "known impactors". Following this, the identified objects would be further analyzed using more conventional methods developed at Leiden Observatory's computational astrophysics group led by Simon Portegies Zwart. Additionally, the developed impact detector could potentially be used as a generally collision detector and repurposed for space debris application or as a research tool to better understand the formation history of the solar system.
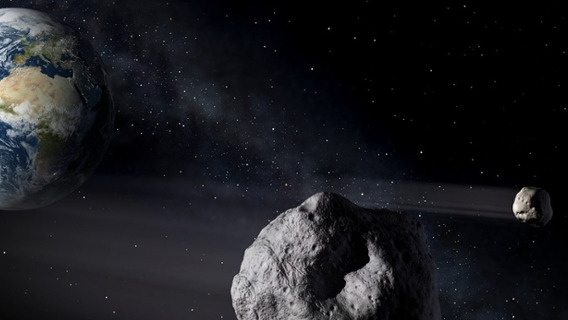