In recent years the Arctic has seen renewed political and economic interest, increased maritime traffic and desire for improved sea ice navigational tools. Despite a rise in digital technology, maps of sea ice concentration used for Arctic maritime operations are still today created by humans manually interpreting radar images. This process is slow with low map release frequency, uncertainties up to 20 % and discrepancies up to 60 %. We propose utilizing emerging Artificial Intelligence (AI) image classification techniques to automate this process, drastically changing navigation in the Arctic seas, with better resolution, accuracy, release frequency and coverage. The AI Convolutional Neural Network (CNN) has proven capable of image classification by generating feature maps and choosing the optimal combination. Research on how best to utilize CNN to solve this is needed, but undeniably the next step for sea ice products, with potential for applying more powerful AI techniques in the future. Automatic sea ice concentration classification is a novel foundation enabling other sea ice products such as sea ice thickness and improved Arctic climate change monitoring. The proposed research project is building on the AI4ARCTIC ESA initiative and the unique and novel sea ice AI4ARCTIC AI ready data set. It comprises 466 overlapping Sentinel-1 radar scenes, radiometer data and sea ice maps produced by the Danish Meteorological Institute and cover the coasts of Greenland. This will be used for training and evaluating algorithms. An obstacle is the high variability of the radar signatures of the sea ice surface for different ice types and formation history and wind-roughened seas may look similar. To reduce the ambiguities with wind roughened seas, wind speed estimates from the European Centre for Medium-Range Weather Forecasts (ECMWF) will be included. This study will develop novel CNN architectures to do data fusion between dual polarized radar, radiometer and wind speed data.
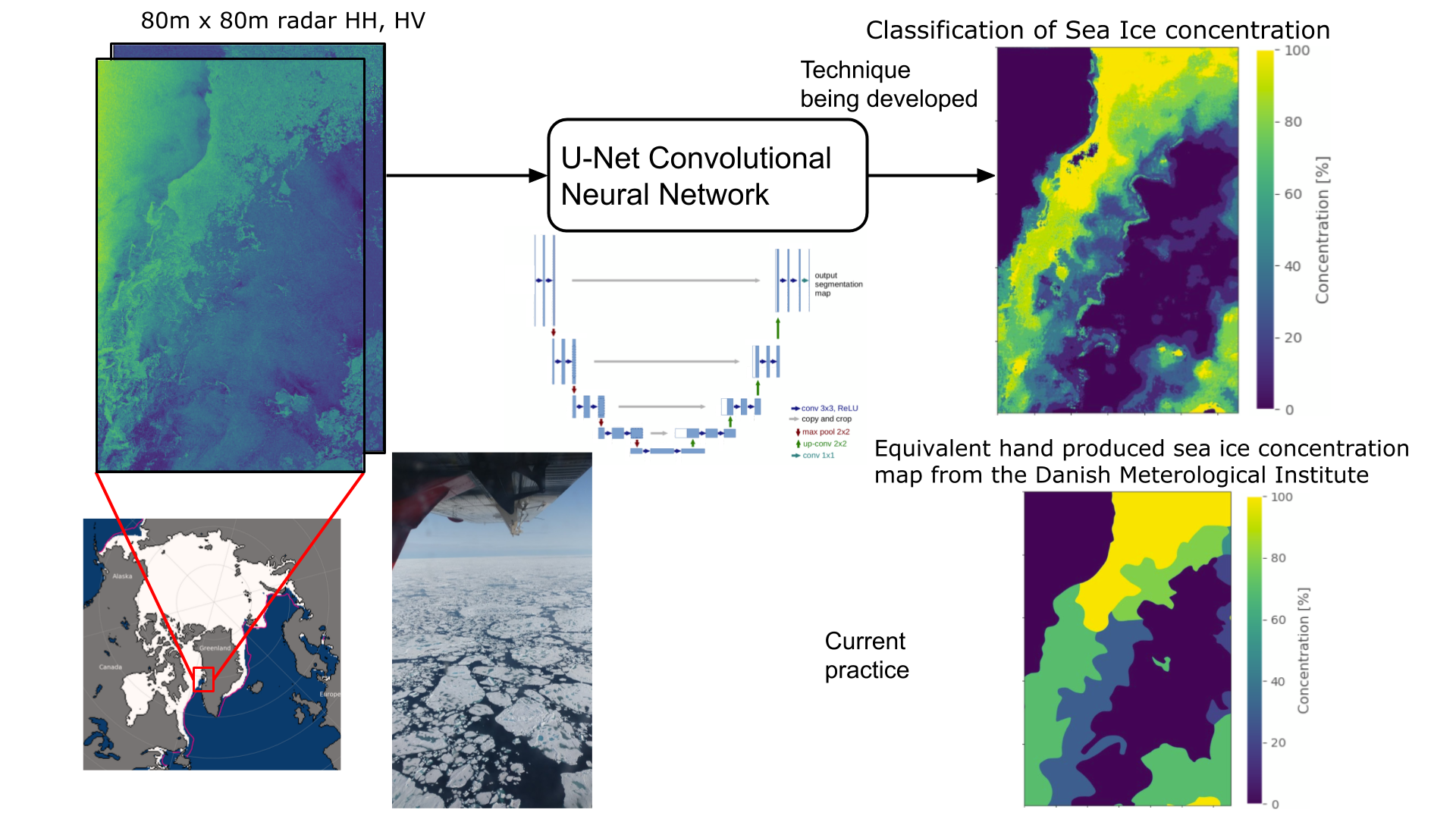