Severe requirements imposed by space applications can be only satisfied by the most sophisticated material systems, which often derive their unique properties from combining different constituents (composites) or carefully engineered micro and macro architectures (metamaterials). The amount of possibilities translates onto an enormous design space, which can be explored only with an efficient computational approach. Recently, data-driven approaches to design are showing great promise in discovering new materials and structures with unprecedented properties. For example, a data-driven framework relying on a new Bayesian machine learning method (sparse Gaussian processes) was used to create a metamaterial with super-compressibility, despite being manufactured with brittle base materials. Additionally, machine learning allows to account for material imperfections, enabling robustness of the designs. However, the framework suffers from the "curse of dimensionality": each new design variable implies an exponential growth of the design space, which for more complex design problems results in prohibitive computational cost. Those limitations could be surpassed with quantum computing - by replacing the machine learning step with an exponentially faster quantum algorithm for Gaussian processes (QGP). However, the algorithm requires a large fault-tolerant quantum computer, which is still decades ahead. We propose to explore enhancing the data-driven framework with noisy intermediate-scale quantum devices (NISQ) available within this decade (e.g. by tailoring the QGP concept to NISQ specifications). The approach provides an alternative for applying quantum computing in materials science, which enables design of material at all length-scales. If successful, the resulting quantum-enhanced framework will surpass the classical limitations ultimately enabling the computational approach to design materials with unprecedented performance - a key necessity for the space industry.
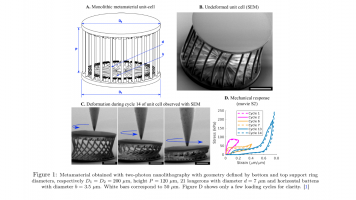