Duration: 12 months
Next-generation SAR systems will offer a giant leap in performance, coverage and revisit times, leading to the generation of very large volumes of data. An effective quantization of SAR raw data becomes of utmost importance and, in this scenario, artificial intelligence represents a most promising tool for tackling the challenge of data rate optimization. Starting point is a preliminary proof-of-concept for an adaptive onboard quantization of SAR raw data based on the dynamic estimation of two-dimensional bitrate maps using a trained deep learning (DL) architecture. The bitrate estimation task is treated as a supervised regression problem which aims at directly linking the information content of the SAR raw and focused data domains using DL, without the need for on-board processing.
In order to achieve a robust and generalized DL architecture, we need to tackle the model design and optimization by considering a global SAR mission acquisition scenario. We will use experimental uncompressed TanDEM-X SAR acquisitions to design an extended training and testing dataset, yielding the generation of adaptive bitrate maps to be used for onboard SAR raw data quantization. We will generate synthetic SAR raw data at global scale, which will allow for performing hyperparameter tuning of the DL model with respect to a certain data rate requirement. The obtained raw data will be used to assess the required data rate over selected orbits, with the goal of achieving a robust, joint optimization of both the data rate and the resulting performance of SAR and InSAR products. We will estimate the overall quantization performance and data volume, and benchmark the proposed method with respect to state-of-the-art quantization algorithms.
In view of a potential onboard implementation, we will conduct a hardware feasibility assessment of the proposed DL-based compression scheme, investigating the requirements, limitations and challenges given by the current available technology.
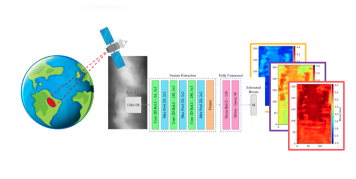