
Closed
Duration: 36 months
The rapid expansion and increasing complexity of data collected by astronomical observatories are outpacing the capabilities of traditional data processing techniques. While conventional methods have been foundational in transforming images into scientifically usable information, there is a growing need for more efficient methods capable of managing terabytes of nightly data. To bridge this critical gap, we propose the conceptualisation and development of the first Machine Learning (ML) telescope data processing pipeline, along with a comprehensive evaluation of the set of scientific discoveries that quicker processing and improved sensitivity at low signal-to-noise ratio would bring to time-domain astronomy. This project will leverage the four telescopes of the Asteroid Terrestrial-Impact Last Alert System (ATLAS) as a testbed, targeting the system's existing challenges, including lengthy data processing times and a high rate of false positives. Successfully demonstrating the effectiveness of our ML solution, we aim to establish a scalable method applicable to ground-based telescope networks, indirectly benefiting entities reliant on this data, such as ESA's Near-Earth Object Coordination Centre (NEOCC). Additionally, we plan to evaluate our pipeline's adaptability to space-based images by comparing its components with the processing techniques used in EUCLID and planned for ARRAKIHS. The project will begin with the creation of a comprehensive training dataset (publicly released), combining labelled synthetic data generated with ESA's Pyxel simulator and actual observational data from ATLAS. This dataset will train pre-developed ML models to perform tasks such as source localisation, feature extraction, star-galaxy classification, and transient detection. Emphasizing a modular architecture informed by ML Operations (MLOps) principles, our pipeline will be designed for adaptability and continuous improvement, setting a new standard for data processing in astronomy.
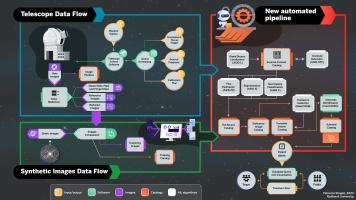