Duration: 24 months
Low-cost and low-power accelerators for Machine Learning (ML) execution have recently been introduced on the market. By optimizing the data transfer and relying on dedicated functional units design, ML accelerators efficiency outperforms traditional computing devices. The power efficiency of ML accelerators can potentially enable the use of ML even in power-constraint small or nano satellites, institutional missions, and NewSpace applications that go from image processing and debrits detention, to segmentation and cloud screening. The goal of this project is to characterize the reliability and improve the availability of the novel commercial Adaptive Compute Acceleration Platforms (ACAPs) embedding a ML accelerator. Because of the unprecedented complexity of the heterogeneous architecture and of the software application, such a study has never been done before. We target the Xilinx EdgeAI Versal VE2302, that embeds the AI Core AIE, since various companies are currently evaluating it for future space systems and it is expected that the device will be proposed for both institutional missions and commercial satellites; however, questions about SEE effect remain. We exploit the synergy between expertise from material sciences, radiation reliability, computing architecture, and robotics to provide a thoughtful radiation response of the component. We will proceed with a bottom up reliability and availability study. First, we will perform experiments to measure the latch-up response of the VE2302. Then, we will characterize the availability of the VE2302 components by measuring the single event data corruptions and functional interrupts probabilities under operative mission conditions and running OBPMark-ML payload. Finally, we will perform ML specific application-level tests to identify the errors that cause critical misbehaviours and propose innovative solutions to increase the device availability by adding dedicated (software) redundancy to mask or remove errors.
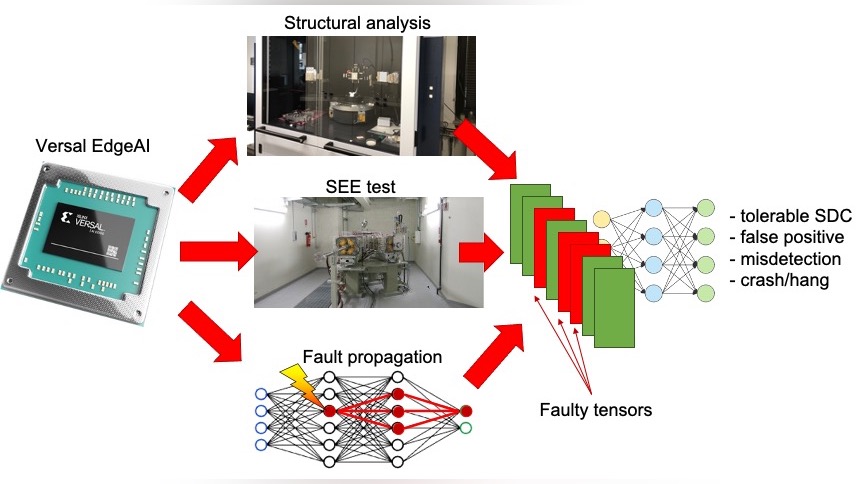