Duration: 36 months
In Orbit Servicing (IOS) will revolutionize space exploitation, transforming how we interact with orbiting objects. This shift will mitigate the space debris issue, enable satellite reparations, and influence future spacecraft (S/C) designs. IOS considers a Chaser S/C approaching and interacting with a Target S/C in close proximity, often with limited knowledge of the Target. The Chaser relies on its Guidance, Navigation, and Control system to perceive the environment, plan the relative trajectory and maneuvers, and execute them with the available hardware units. Relative navigation is a key enabling function for autonomous proximity operations as the Chaser needs to autonomously localize itself with respect to the Target. State-of-the-art vision-based navigation (VBN) solutions rely on passive cameras and dedicated image processing algorithms to extract navigation information. The use of data-driven/AI approaches for VBN is gaining attention in the last years as they have a lower computational burden when compared to classical techniques. Unfortunately, because of their low level of maturity, their validation remains a key open research point. Moreover, the robustness of AI-based solutions to uncertainties in the target's shape and optical properties has never been addressed. Therefore, this research aims at designing, testing, and validating a novel robust and implementable navigation architecture that combines model-based filtering techniques and AI-based image processing. A co-funded PhD among the European Space Agency, Thales Alenia Space and Politecnico di Milano is proposed to fulfill these goals. Experimental activities are foreseen to demonstrate the embeddability through processor- and camera-in-the-loop tests on optical and robotic facilities. The outcomes of this research project will provide a potentially disrupting and enabling navigation technology for future IOS missions.
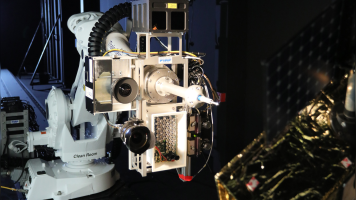