Duration: 36 months
Given the increased space traffic, the number of objects re-entry every day is going to increase as well. However, predicting the outcome of the re-entry process (demise, fragmentation and possible landing points) is challenging because of 1) the complex physical interdependence of structural, thermal, and fluid dynamics, and 2) the multiple related sources of uncertainty in the modelling and simulation of the re-entry process.
This project proposes a novel approach to the robust and rapid prediction of space object re-entry. By considering both uncertainties in initial data and model fidelity, we will develop both a forward uncertainty propagation and backward uncertainty model updating across the whole re-entry trajectory. For the forward step, we propose a novel propagation method that extends the High-Dimensional Model Representation (HDMR), developed in [1,2], to handle both aleatory and epistemic uncertainty in the form of P-boxes [3]. Uncertainty modelling with p-boxes is an imprecise probability technique that allows a unified treatment of both aleatory and epistemic uncertainties in the same framework. Backwards, or inverse, uncertainty model updating [4], will be used to reduce the epistemic uncertainty, in the model and initial conditions, from data. A multi-fidelity modelling approach will be implemented to achieve a balance between precision and efficiency. The difference between high- and low-fidelity models will be treated as an epistemic uncertainty and reduced via model updating with a Bayesian Markov chain Monte Carlo approach [5].
____
[1] A. Falchi, E. Minisci, M. Kubicek, M. Vasile, S. Lemmens, HDMR-Based Sensitivity Analysis and Uncertainty Quantification of GOCE Aerodynamics Using DSMC BT - Stardust Final Conference, in: M. Vasile, E. Minisci, L. Summerer, P. McGinty (Eds.), Springer International Publishing, Cham, 2018: pp. 301–323.
[2] P. Wei, J. Song, S. Bi, M. Broggi, M. Beer, Z. Lu, Z. Yue, Non-intrusive stochastic analysis with parameterized imprecise probability models: I. Performance estimation, Mech. Syst. Signal Process. 124 (2019) 349–368. https://doi.org/10.1016/j.ymssp.2019.01.058.
[3] S. Bi, M. Broggi, P. Wei, M. Beer, The Bhattacharyya distance: Enriching the P-box in stochastic sensitivity analysis, Mech. Syst. Signal Process. 129 (2019) 265–281. https://doi.org/10.1016/j.ymssp.2019.04.035.
[4] S. Bi, M. Broggi, M. Beer, The role of the Bhattacharyya distance in stochastic model updating, Mech. Syst. Signal Process. 117 (2019) 437–452. https://doi.org/10.1016/j.ymssp.2018.08.017.
[5] S. Bi, K. He, Y. Zhao, D. Moens, M. Beer, J. Zhang, Towards the NASA UQ Challenge 2019: Systematically forward and inverse approaches for uncertainty propagation and quantification, Mech. Syst. Signal Process. 165 (2022) 108387. https://doi.org/10.1016/j.ymssp.2021.108387.
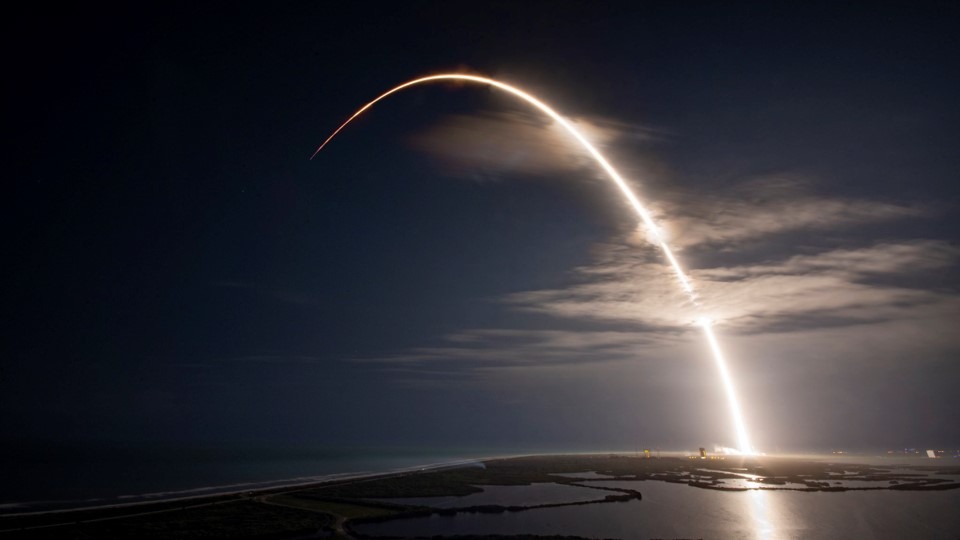