Duration: 12 months
OrbitGPT aims at developing a large orbit model (a Foundation Model) that enables generative astrodynamics. The idea behind generative astrodynamics is to generate trajectories with desired features without running expensive design or orbit determination algorithms. For example, for a new mission, one may want to generate different possible multi-gravity assist trajectories, or alternative periodic orbits in an n-body model. The utility of a Foundation Model (FM) sits in its generality and applicability to multiple specific cases to return orbits and/or derived quantities without the need of orbit propagation and the solution of potentially expensive optimisation problems. More applications are possible via transfer learning by retraining the model with additional data. For example generating Conjunction Data Messages, non-observable parts of a trajectory, or regular and chaotic motions in a cartography. Such a generative model can be inserted in adversarial architectures to discriminate anomalies, like manoeuvres or unexpected variations in natural dynamics, or can be used to train other models on new synthetic data. The literature already presents previous works on the generation of large databases to train machine learning models specialised in optimal control for low-thrust trajectories, landing trajectories and conjunction events (just to name three examples). The authors already developed a proof of concept of a self-supervised learning based orbit model, called ORBERT, that is a precursor of an OrbitGPT architecture. In parallel the authors are developing a more general generative model for dynamical systems. A generative astrodynamics model would be a subset of the models developed in the context of general generative dynamic models but specialised in orbital mechanics and derived quantities of interest.
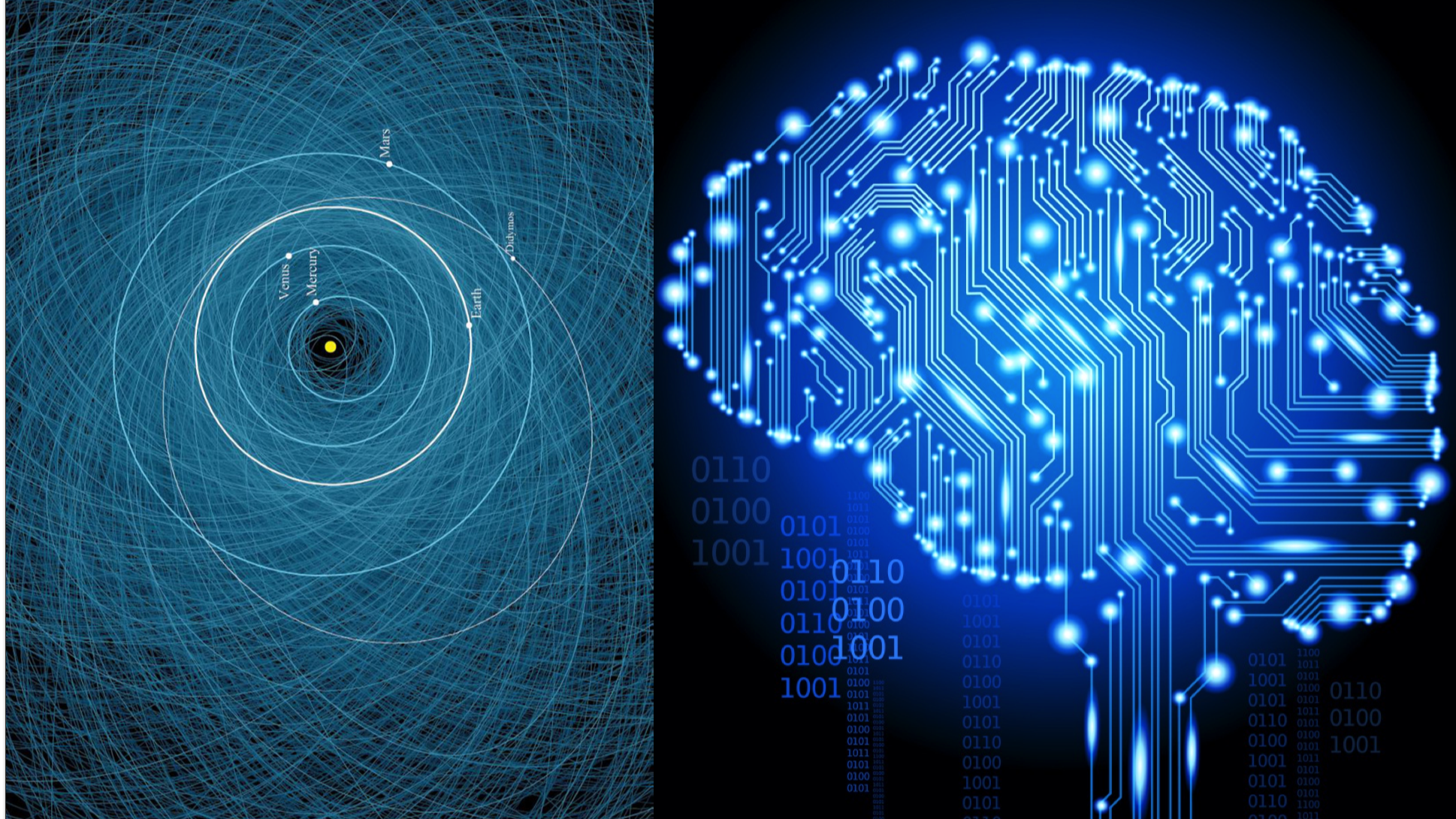