Current technological advancements bring exciting opportunities in hyperspectral image (HSI) analysis across various Earth observation (EO) applications. To extract value from such highly dimensional data, an array of machine learning (ML) pipelines emerged. Although the world is not “labeled” and capturing ground truth (GT) collocated with HSI is costly and not scalable, a plethora of supervised methods were proposed for EO downstream tasks. There are indeed approaches for dealing with limited GT, and they include transfer, semi-, and self-supervised learning, together with HSI data augmentation.
In some cases, only coarse-grained GT is available, e.g., due to low spatial resolution of airborne/satellite imagery and/or specific in-situ measurements, with soil parameters’ estimation being a prominent example here. In this task, soil samples are collected in the field using e.g., a Zig-Zag strategy. They are mixed together & forwarded to specialized labs for chemical analysis. Thus, GT constitutes N soil parameters obtained for S samples collected for each field. Such weakly-labeled image-level GT is assigned to the HSI of a field, being hundreds (or even thousands) of pixels. Since the number of in-field sampling points is limited & scattered across large areas, image-label GT tuples are ultimately assigned to heterogeneous (and likely noisy) pixels – it is obvious that not all pixels contribute equally to lab-confirmed parameters.
In this project, we tackle the challenge of building supervised classic and deep ML models from weakly-labeled datasets in both regression and multi-label classification. Our main objective is to develop new algorithms for this task and thoroughly validate them over our real-life soil analysis dataset. We hope that the project will be an important step toward building well-generalizing AI models from image-level GT labels which are much easier & cheaper to obtain in real EO scenarios.
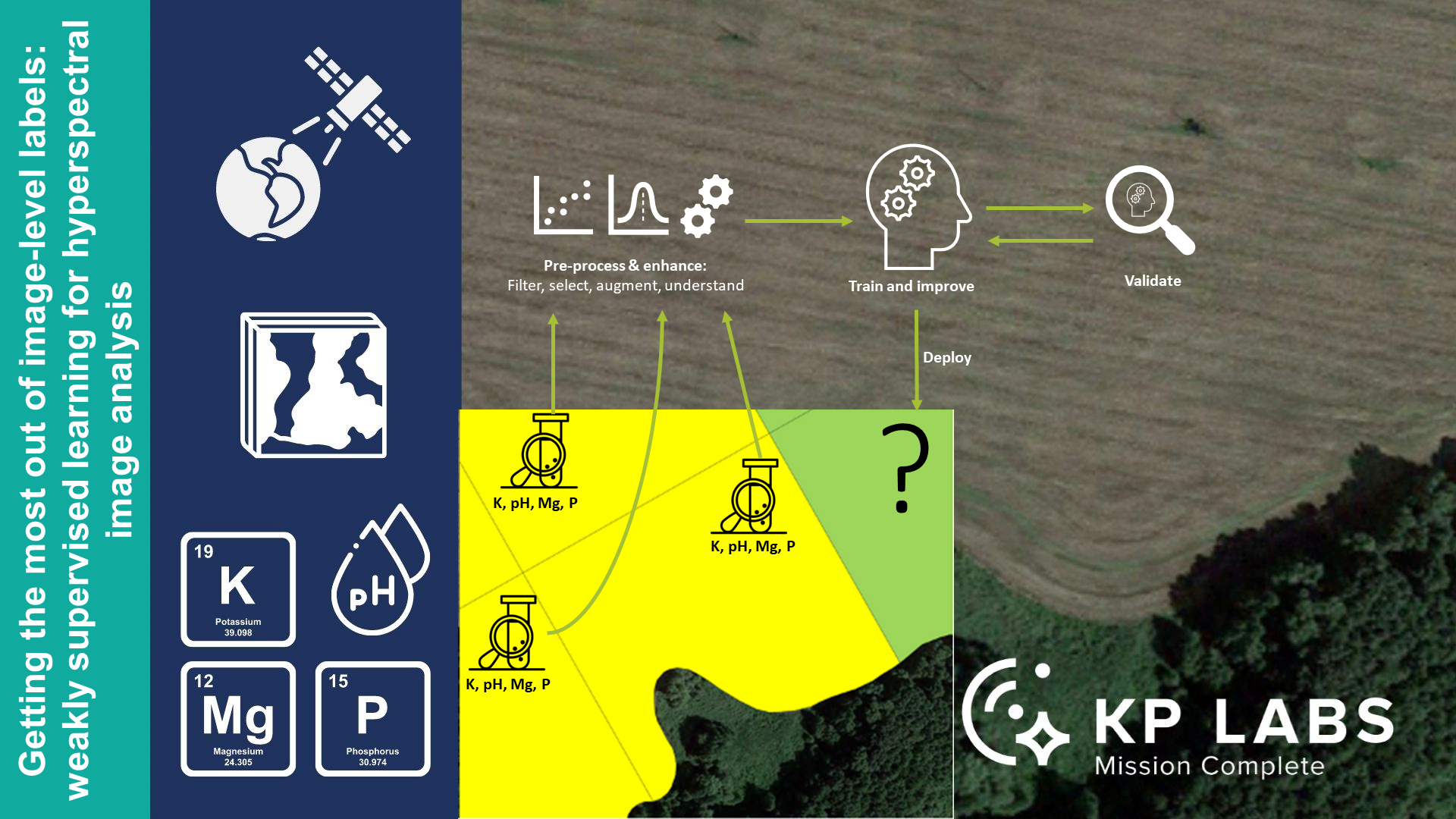