Duration: 36 months
The accurate modelling of material processes, structures and properties in Advanced Manufacturing requires predictive transport parameters such as multiphase flow coefficients as well as grain growth rates, tensile strength and fatigue. These coefficients are needed over a range of operating conditions for spacecraft deployments in outer space and in planetary atmospheres. Our aim is to extract accurate material transport and strength coefficients from time-series data (obtained from high-fidelity simulations) to accelerate the design of experiments for Integrated Computational Materials Engineering (ICME). To elucidate the approach let us consider the case of thermal conduction. While in ordinary gases it is the collisions between nearby particles that enforce a local energy flow from hotter to colder regions, under extreme conditions, heat conduction is no more mediated by nearby collisions instead complex kinetic, radiation and chemical effects appear at work. Several modified versions of Fick's law as well as empirical formulas based on fits to experimental data have been proposed, but they are often poor and fail to generalize. This applies to many other transport and material coefficients. Here we want to propose a novel machine learning approach to address the complex micro-physics of material strength properties and to identify their emergent behaviour via closed mathematical expressions. This is done by using a Graph Neural Network (GNN) to represent the discrete description of the underlying continuum system and then applying deep learning techniques to obtain a representation of the material strength process as a function of the state variables. The latent representation learned by the GNN is then extracted with a symbolic regression analysis. Our long-term goal is the development of augmented methods to ultimately improve the design and verification of new spacecraft components, as well as help to reduce their development time and cost.
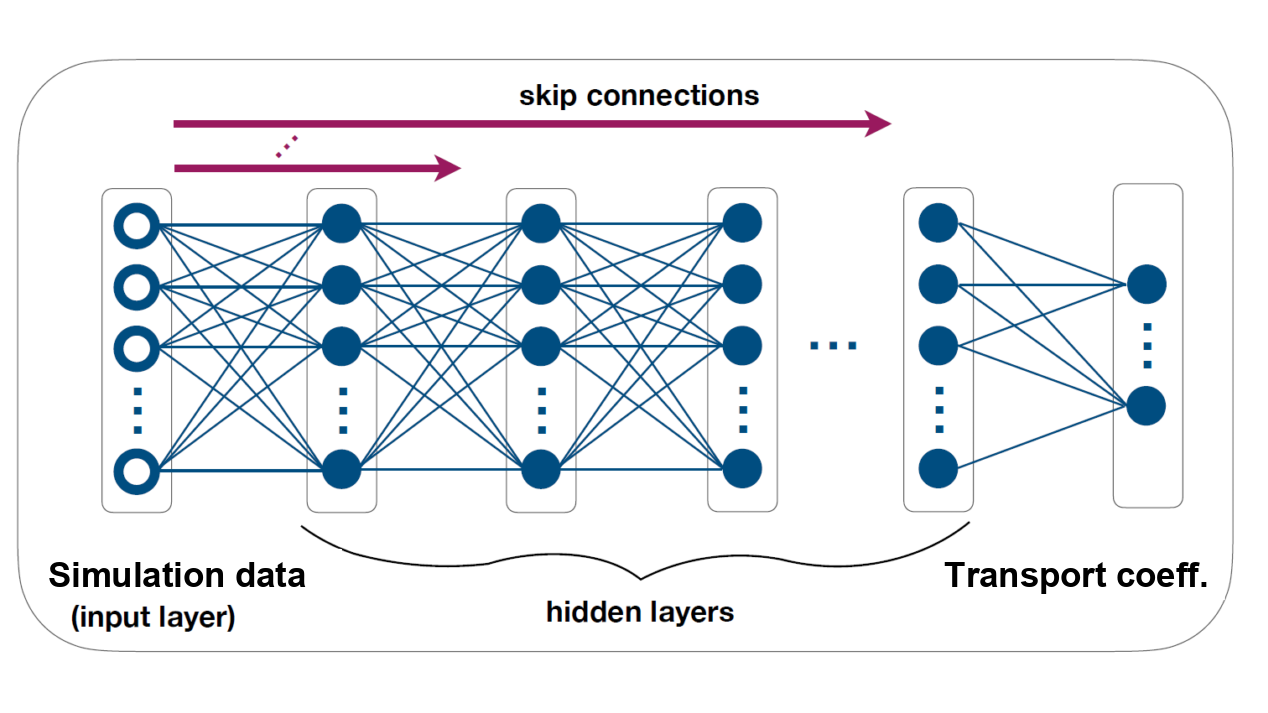