Duration: 36 months
Computer vision is becoming prominent in the space industry. It is an enabler for complex missions: probes to Solar System objects or autonomous in-orbit rendezvous missions. Vision-based navigation integrates imaging sensors and computer vision algorithms in functional avionics system for missions like JUICE, MSR ERO, ATV or future CLTV and EL3. Validation relies on test datasets: computer generated images, real data from test benches or recordings of previous missions. The datasets are subject to possible flaws and their representativeness is often debated. There is no consensus on a validation methodology for them. For advanced project phases, validation is a costly task because it relies on highly detailed modelling and analysis, especially in our teams of Airbus and ESTEC. For example, suppose you want to embed an algorithm relying on edge detection to navigate relative to a satellite. Do you need to model material properties in details? Now what if the algorithm relies on 3D reconstruction? There is certainly a trade-off between the model complexity and the expected gain on Image Processing (IP) performance. How complex must a simulation be to validate an IP solution? Conversely, can the algorithm serve as a metrics to specify a simulator? These questions are largely unexplored in the literature especially for the specific conditions of space. For this PhD project we propose to adapt to the space sector knowledge from academia in Computer Vision. The researcher will develop a methodology to serve as guideline for the qualification of future image simulation environments. In practice the work will consist in assembling datasets for various mission profiles (simulation / test bench / archival images), developing mathematical metrics to compare their geometric and radiometric properties and analyzing the impact on performances for various algorithms. The work carried out can be quickly put in practice by closing gaps in the design and development of ESA missions.
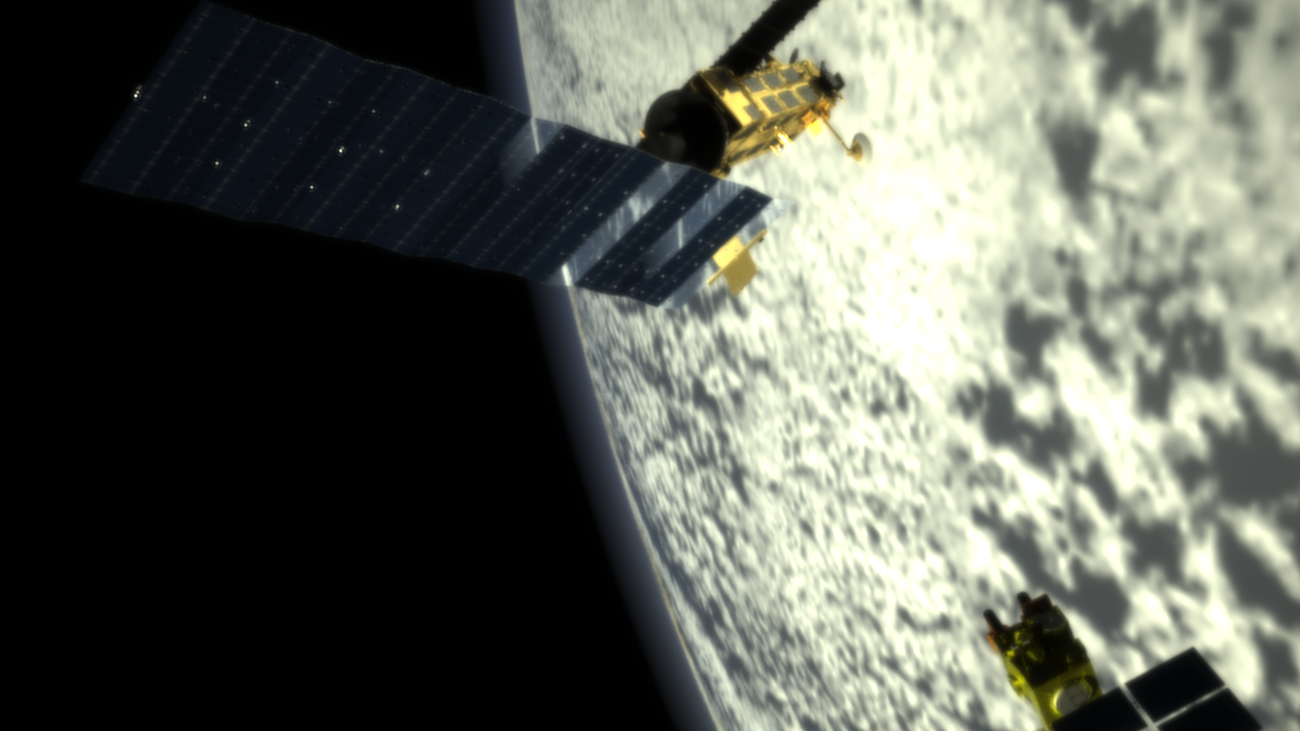