Future space missions, e.g., manned exploration, reusable space transportation, in-orbit servicing, or distributed flight, must rely heavily on self-awareness, adaption and autonomy. Complex sensor-fusion provides the high-degree of self-awareness assuring robust and precise localisation and attitude determination. Novel intelligent (e.g., event-based) camera systems together with inertial sensing devices permit robust perception enabled planning and decision-making [1]. However, classic guidance, navigation and control methods are not sufficient for safe self-aware and adaptable operations.
Hence, a rethought, mathematically well-founded approach is required combining model-based and data-driven embedded optimisation for safe autonomy. Real-time system identification (SysID) is key for online modelling and decision-making in dynamically changing environments. Current research aims at developing design frameworks combining online learning [2,3] and robust data driven synthesis [4]. Today this process is fully offline, i.e., the modelling, SysID and control design, rely on risk-constrained offline learning [5,6], low-order uncertainty models (linear fractional transformations (LFTs)) and offline identification [7], whereas online robust autonomy [8] is less explored.
Thus, the project develops a real-time embedded optimisation methods for robust modelling, analysis, planning, and decision-making. Through artificial intelligence (AI), the real time SysID and dynamical modelling process will be coupled to a low-order LFT structured uncertainty model generation. These provide models for robust control synthesis. Contraction theory and integral quadratic constraint will be used for online robustness analysis. Subsequently, the control law is optimised online and updated in real-time using the latest acquired system dynamics. The applicability will be shown on existing ESA testbeds. Summarising, an AI-enhanced robust SysID, modelling and synthesis framework is provided.
__________
[1] Dean, S., Matni, N., Recht, B., Ye, V., Proceedings of the 2nd Conference on Learning for Dynamics and Control, PMLR 120:350-360, 2020, Online: http://proceedings.mlr.press/v120/dean20a/dean20a.pdf
[2] Harrison, J., Sharma, A., Finn, C., & Pavone, M., Continuous meta-learning without tasks. Advances in neural information processing systems, 33, 17571-17581, 2020, Online: https://proceedings.neurips.cc/paper/2020/file/cc3f5463bc4d26bc38eadc8bcffbc654-Paper.pdf
[3] Harrison, J., Sharma, A., Calandra, R., & Pavone, M., Control adaptation via meta-learning dynamics. In Workshop on Meta-Learning at NeurIPS (Vol. 2018), 2018, Online: http://metalearning.ml/2018/papers/metalearn2018_paper58.pdf
[4] Xue, A., & Matni, N., Data-driven system level synthesis. In Learning for Dynamics and Control (pp. 189-200), PMLR, 2020, Online: http://proceedings.mlr.press/v144/xue21a/xue21a.pdf
[5] Chow, Y., Ghavamzadeh, M., Janson, L., & Pavone, M., Risk-constrained reinforcement learning with percentile risk criteria. The Journal of Machine Learning Research, 18(1), 6070-6120, 2017, Online: https://www.jmlr.org/papers/volume18/15-636/15-636.pdf
[6] Chapman, M. P., Lacotte, J., Tamar, A., Lee, D., Smith, K. M., Cheng, V., ... & Tomlin, C. J., A risk-sensitive finite-time reachability approach for safety of stochastic dynamic systems. In 2019 American Control Conference (ACC) (pp. 2958-2963). IEEE, 2019, DOI: 10.23919/ACC.2019.8815169, Online: https://ieeexplore.ieee.org/stamp/stamp.jsp?arnumber=8815169
[7] Schoukens, M., Tóth, R., On the initialization of nonlinear LFR model identification with the best linear approximation. IFAC-PapersOnLine, 53(2), 310-315, 2020, DOI: 10.1016/j.ifacol.2020.12.142
Online: https://www.sciencedirect.com/science/article/pii/S2405896320304006
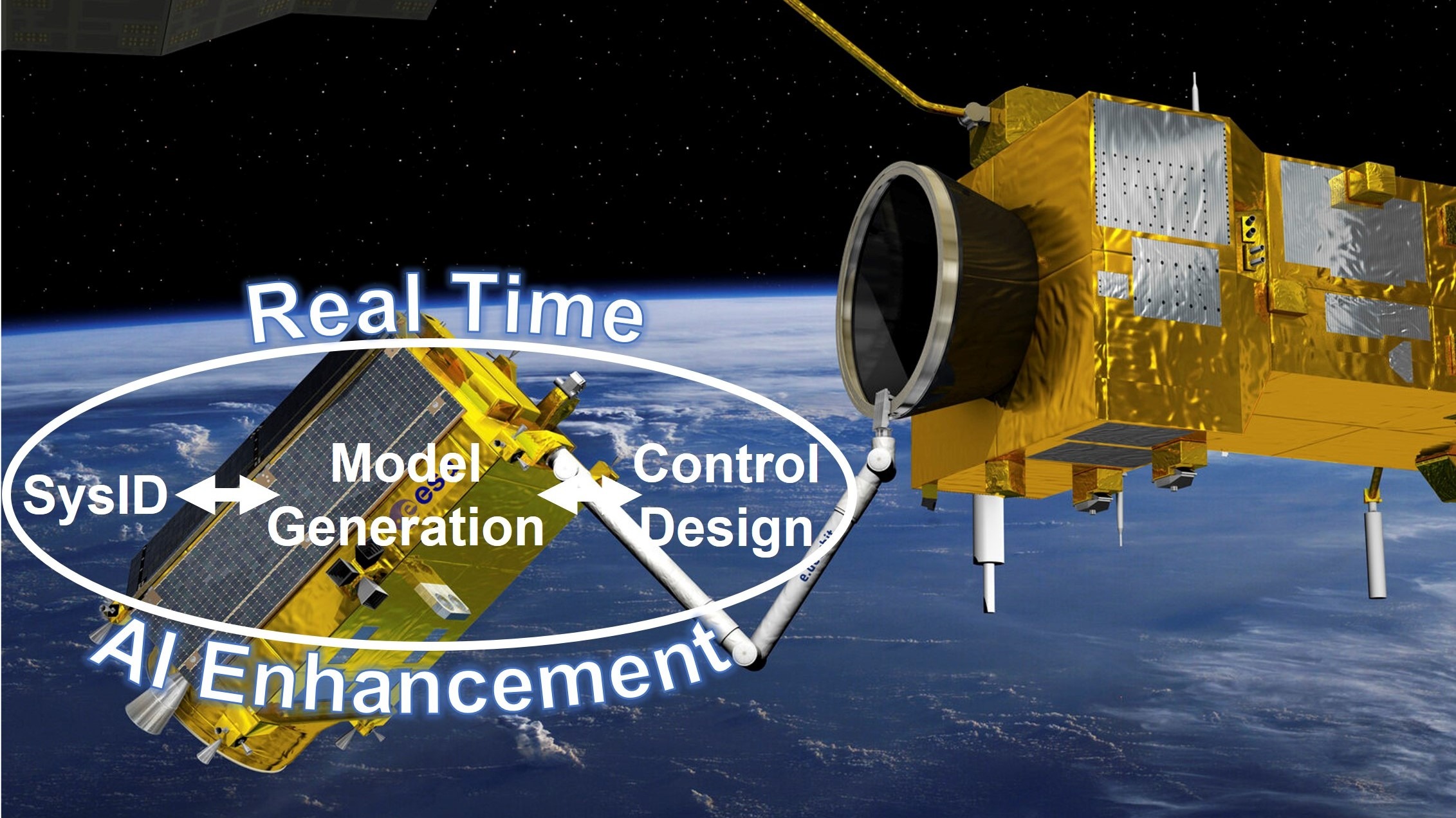