Ensuring sustainable utilisation of space is a priority that has led to substantial investments in reducing the volume of existing debris and improving the design for demise of new spacecraft. Making accurate and fast predictions of the demise phase from the entry interface until ground is at the same time a key enabler and a major challenge in achieving space sustainability. Accuracy and speed will allow identifying reliably de-orbiting conditions that minimise the ground impact risk and will also allow for improved design by reliably assessing the demise performance of new spacecraft. Simulating re-entry scenarios is a demanding task that requires the modelling of aerothermodynamics, structural and flight dynamics in presence of shock waves interaction and structural failure due to severe thermomechanical loads. Existing fast low-fidelity methods have shown reliability issues in simulating the re-entry environment. High-fidelity methods are not yet at the stage where they can be employed without leading to substantial costs in terms of time and effort required to get an answer. DECODE aims at introducing beyond-state-of-the-art data-driven and machine-learning based Reduced Order Modelling (ROM) for the simulation of the aerothermodynamics and structural mechanics during destructive re-entry. Being trained on existing data incorporating a detailed representation of the physics, the proposed ROMs can achieve accuracy and reliability comparable to high-fidelity methods but at a fraction of the time required by high-fidelity since only the ROM model is solved during the simulation of the re-entry path. Non-linear, intrusive and non-intrusive ROM will be implemented to address the complexities resulting from shock waves and fragmentation. DECODE will generate and demonstrate cutting-edge data-driven ROM for the simulation of destructive re-entry leading to more informed decisions than what currently possible given the limits of existing low- and high-fidelity methods.
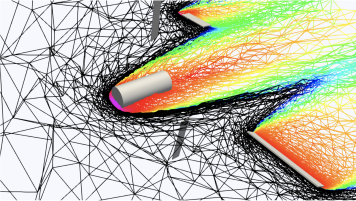