Autonomous tracking of space objects using low-profile hardware is of interest in the context of deep space motion imaging [1] with applications like active debris removal (ADR) [2]. One important aspect of such tasks is the precise position and orientation estimation of objects in space, which has been addressed within two ESA challenges using individual images only [3]. However, for ADR a complete tracking approach has to be realized i.e., information of subsequent detections must be merged to obtain a robust target localization estimation across the different phases of the chasing manoeuvrer. During these phases the target appears in very different distances and positions as well as with time-varying orientation as seen from the chaser. Changing image backgrounds and a wide range of illumination conditions must be tolerated in this process. An autonomous tracking system (auto-tracker) should adapt in real-time to these changing conditions and thereby optimize the image quality for the subsequent detection process. This can only be achieved with a processing unit being closely coupled to the image capturing device or, in other words, with an “intelligent camera”. Therefore, the main objective of this Study is to proof [or disproof] the concept of an "auto-tracker using a field-programmable gate-array (FPGA)-based digital-zoom camera", which fulfils requirements of spaceflight applications with respect to real-time operation and computational resources. The following challenges associated with such auto-tracker shall be addressed in this Study: (1) hardware-aware optimization and quantization of machine learning algorithms suitable for the task addressed in [3] and their efficient implementation in a spaceflight-qualified FPGA; (2) development of a real-time closed-loop algorithm between the processing unit and the image sensor; and (3) use of an animation rendering engine to mitigate the domain gap problem associated with synthetic images.
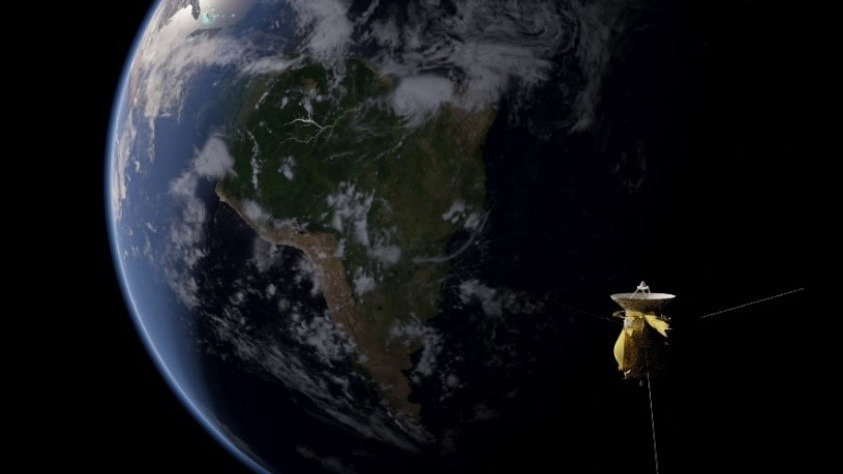