Artificial intelligence (AI), in the form of deep neural networks, is a key enabler for space mission autonomy, performance, and functionality. For remote sensing satellites neural networks are well-suited to deliver computer vision solutions using autonomous observation and data-filtering [1, 2] that enable human operators to maximize use of limited bandwidth. A crucial factor in the successful implementation of deep neural networks on space platforms is the embedded nature of such systems. The performance of onboard space processors lags that of their terrestrial counterparts due to the additional effort required to make circuitry that can operate in extreme radiation, thermal, and vacuum conditions [3]. The spaceborne processors available in the OPS-SAT Satellite Experimental Processing Platform, including the reconfigurable Field Programmable Gate Array (FPGA), address this performance gap and are the ideal test bed to develop new deep learning architectures for future remote sensing and deep space exploration missions. Mission Control proposes using the OPS-SAT platform to characterize a novel FPGA architecture for implementing neural networks on embedded space platforms. FPGAs offer a balance of reconfigurability, generalizability, and utilization efficiency but FPGA deep learning frameworks are still in their infancy [4, 5]. Our FPGA implementation advances how AI can be deployed on spacecraft using the Neural Network Exchange Format (NNEF), an open and standard data format for exchanging information about trained neural networks. We will perform the first comparative study to make use of NNEF for deep learning on a spacecraft by comparing our low-level implementation of the OPS-SAT SmartCam model against the existing high-level model that uses the Tensorflow Lite C API. The software developed as part of our study will improve the operational performance of SmartCam and provide a modular scaffold for future space-based deep learning FPGA technology.
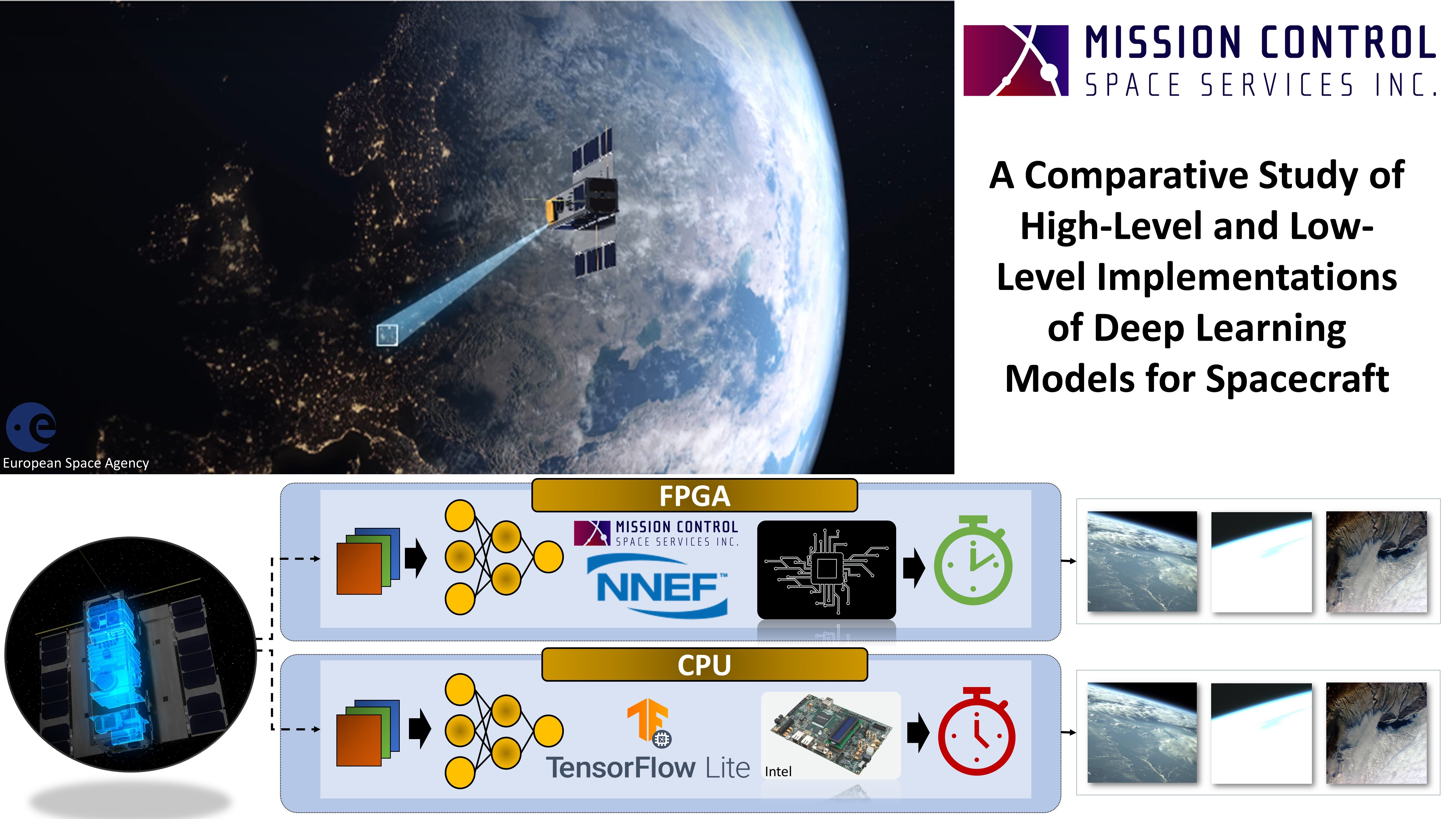