What is life? How unique is this phenomenon to our planet? How common is life in the cosmos? These questions are heavily intertwined. The next cohort of space based telescopes built within our generation will be designed to search for the imprints of biological activity in the atmospheres on planets outside of our solar system. But how do we optimize our telescopes and their observation strategy to maximize our chances to find these signatures? In this project, we will perform timely groundwork towards the observations of potentially habitable worlds - focused on but not limited to the ETH led ESA Voyage 2050 concept LIFE (Large Interferometer For Exoplanets), a space based observatory dedicated to first detect and then to characterize the atmospheric composition of dozens of terrestrial planets around stars in our neighborhood. We are currently working on designing and optimizing the instrumental setup and observation strategy for a mission like LIFE. One of the key elements for this study is atmospheric retrieval, the inverse modeling technique used to derive a planetary atmosphere's structure and composition from a (simulated) observation . At our current design phase of LIFE we need to theoretically reproduce the results of a survey of 100s of planets and retrieve 1000s of modelled measurements coming from our LIFEsim observation simulator. To realize this we need faster and better scalable methods than those currently used, which are both time-consuming and compute-intensive. This problem is pre-destined for a Big Data and ML approach.
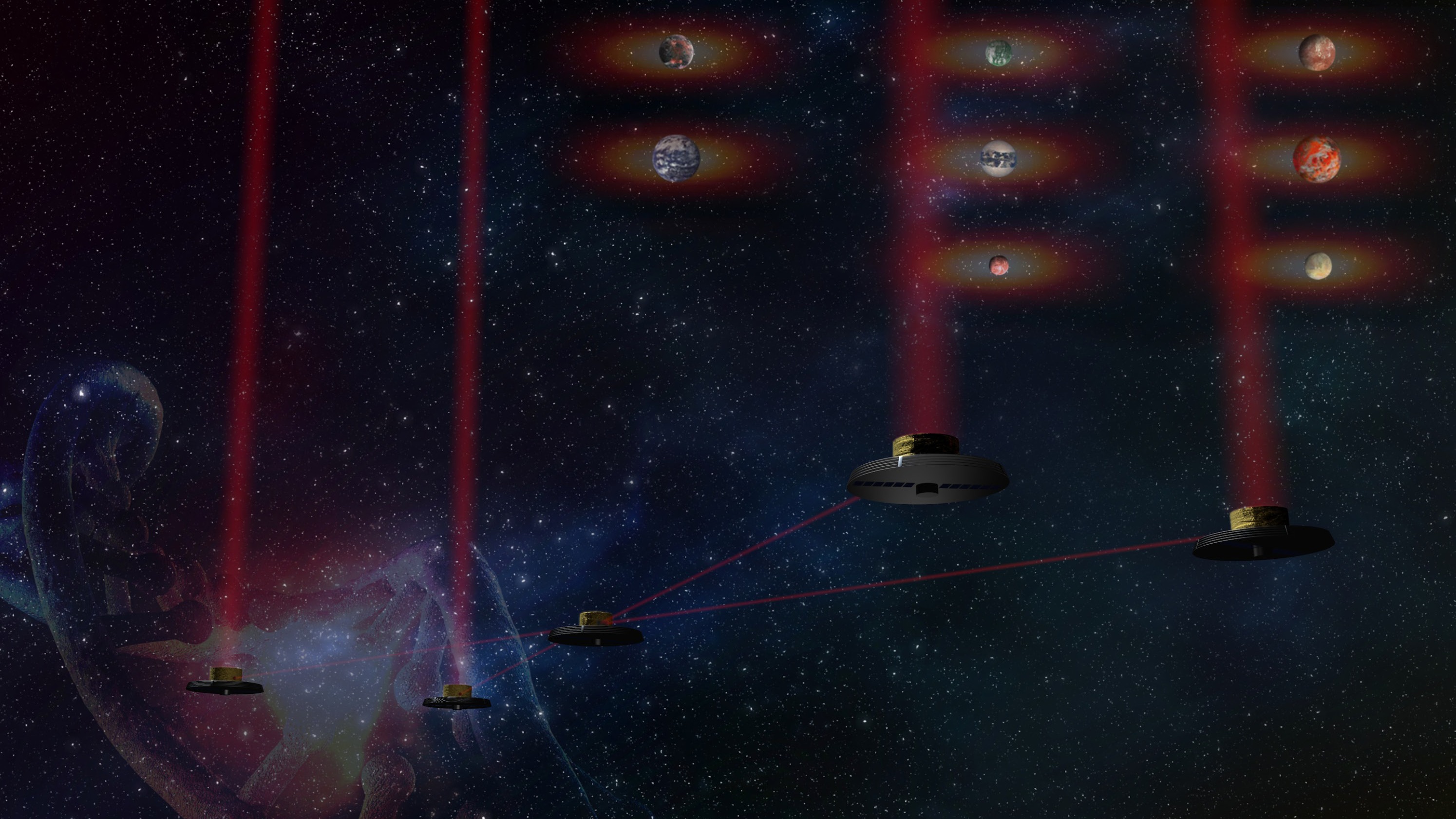