For plastic identification and tracking, we need rich spectral info in hyperspectral (HS) data. Research based on full stretch (0.4 to 2.5 μm) HS for microplastic suggests SWIR ranges are most useful in detecting debris >300 μm (Karlsson et al, 2016). In Garaba and Dierssen (2018), an airborne HS study of synthetic hydrocarbon detection using SWIR absorption features successfully identified debris from marine harvested macro- (>5mm) and microplastics (<5mm). Image analysis on 7.1 m pixel also identified band depth using 1702, 1732 and 1742 nm calibrated at-sensor radiance are most useful. In Driedger et al (2015), the authors suggested it is possible to detect common plastic debris in beach sand using NIR reflectance spectrometry at the range 890–2500 nm. In terms of spatial resolution for identification and tracking, Moy et al (2016) used HD aerial photo (30cm) to track debris. In summary, we want HS data at SWIR (1000-2500 nm) around 0.5 m GSD.
Both of these characteristics are non-existent in current EO missions, and will still be difficult to find in future EO missions. Therefore, we propose (1) to use novel spectral and spatial enhancement method to generate simulated EO HS SWIR from Sentinel 2 MSI using spectral response function modeling, and (2) CNN based 2 branch feature extraction model for capturing detail feature in spatial and spectral domain for plastic debris identification. For a certain MS/HS sensor pairs, spectral responses between different MS/HS image pairs are assumed to be unchangeable despite of different landscapes. In spectral improvement strategy, the relationship between the spectral responses of Sentinel 2 MSI and Hyperion-like HS image can be estimated through auxiliary MS/HS image pairs captured from different landscapes. Transfer learning based 2-branch CNN will be built to perform PANsharpening with a HD panchromatic or SAR image. The result is a multi-model based synthetic HS data most suitable for plastic debris identification.
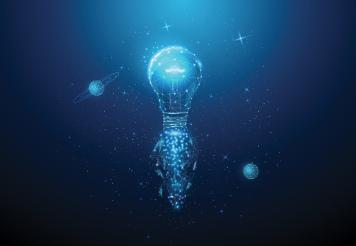