Tables and graphs are knowingly used to organise data within a company with different level of depth and complexity, knowledge graphs are the natural way to model such data when semantics is added to it. Knowledge graphs are particularly useful because they can cope with data diversity (high-quality complete data and sparse and incomplete data), they have a high degree of scalability and flexibility (the semantic data model can be inter-operational, large, wide and as deep as needed) and, last but not least, they provide reasoning and inference capabilities by using semantic standards to describe the structure of the information in the graph. Google mainstreamed knowledge graphs in 2012, since then enterprises have realised that technologies making web search smarter (OWL, SPARQL, RDF, and knowledge graphs) can also be applied to enhance enterprise data linkage, reusability and interpretability. This is why in the last few years a new term has been created: Enterprise Knowledge Graphs (EKG). Alongside the development of the idea itself and its application outside the World Wide Web, digital technologies have emerged to aid the development of custom EKG solutions; to name a few: Stardog, Ontotext, Grakn, AllegroGraph. In the space domain NASA’s Stardog is an example of Knowledge Graph platform built for NASA to manage the relationships that exist not just in the data sources but also among and between them. In order to roll out knowledge graphs in companies, however, more than databases are required. These are the key elements that are necessary for developing a solid knowledge graph for the targeted application: a system engineering taxonomy, a system engineering ontology (entities and relations), system engineering content and data sources (system engineering models) and a knowledge graph modelling framework.
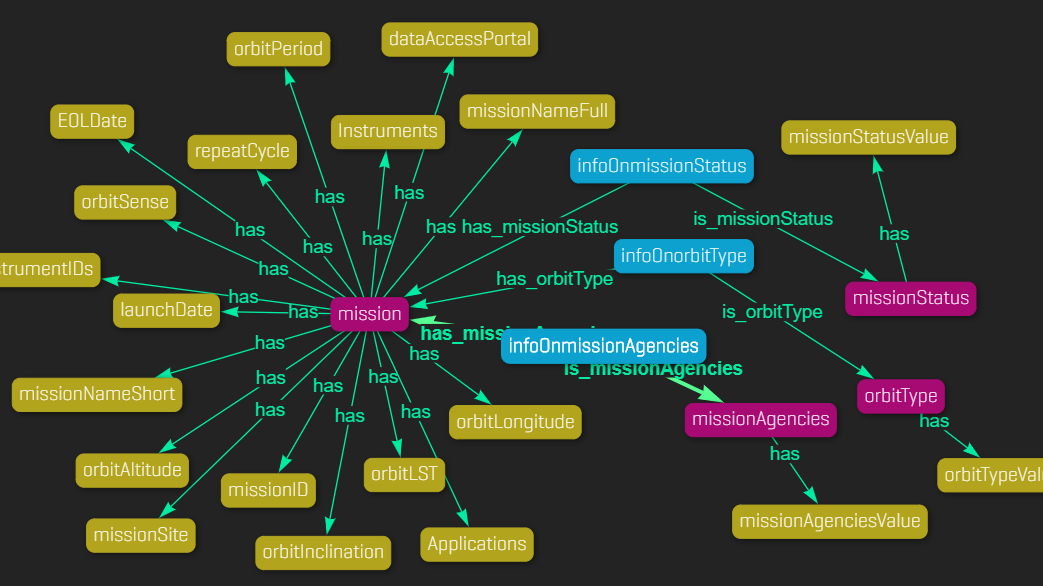