Recent advances in the fields of Big Data and Machine Learning enable the usage of large sets of sensor data coupled with test data to better understand the behaviour of components in operations and predict their failure rates. It is proposed to apply this methodology to better characterize Galileo clock failures, with a particular focus on the Tantalum Capacitor. The aim of the study is to improve the characterization of its failure rate by developing Machine Learning algorithms based on test data and in-orbit data. There are limitations to perform failure-analysis based on in-orbit data, mainly due to a small size of population and the traceability of failures down to component level. An approach is therefore suggested to use the existing data: not only in-orbit operational and sensor data, but also test data at component or equipment level to improve failure rate predictions and identify causes of Galileo clock failures with a particular focus on the Tantalum Capacitor. Applying machine learning for predictive maintenance on the Galileo system, and in particular Galileo clocks, has a high potential to deliver a significant impact. In particular, it leads to a more reliable satellite lifetime characterization and a better constellation maintenance planning for higher system availability. Additionally, it will be an asset for designing and testing the next generation of Galileo satellites. The associated exploitation of space resources and prolongation and risk assessment of mission life can be improved through this approach. The adopted methodology also has good potential towards generalization, allowing a multiplication effect on the reach and impact of the developed research and technology.
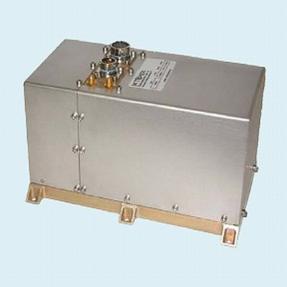