Pipeline networks span multiple thousands of kilometres and need to be monitored on a regular basis to identify incidents and leakages. For oil and gas producing regions, it is of paramount importance to effectively protect communities and the environment [1]. Especially methane (CH4) leakages need to be detected as methane is one of the most potent greenhouse gases. In 2020, about 70 million tons were emitted from oil and gas operations worldwide according to IEA. Nowadays, airborne and in-situ measurements are the main source for detection. Compared to aerial solutions (~100€/km²), only a spaceborne solution is able to provide a more cost-effective (~5-25€/km²) as well as systematic monitoring of the pipelines [2,3]. Currently there are only few publications about spaceborne gas leakage detection for pipelines. They are even not mentioned in a recent monitoring survey [4]. Most publications (e.g. [5,6]) are only using one sensor type as input and therefore do not benefit from complementary information. The main objective of this PhD is to develop and test ML approaches for spaceborne CH4 detection using multiple data sources by employing data fusion and time series (TS) analysis. With the help of data fusion problems like revisit time and partially cloud coverage can be overcome. Time series analysis will be used to integrate temporal information such as the methane plume formation over time. Different data fusion and TS techniques will be elaborated and tested. Another objective is to analyse data sources with respect to their relevance for detection. For this, existing feature extraction algorithms based on DL can be used along with data fusion to combine features from different sources. Lastly, to allow efficient training and to reduce the amount of training data, transfer learning techniques using pre-trained models will be applied. The key novelty of this idea lies in the combination of multimodal and spatio-temporal DL approaches for methane detection.
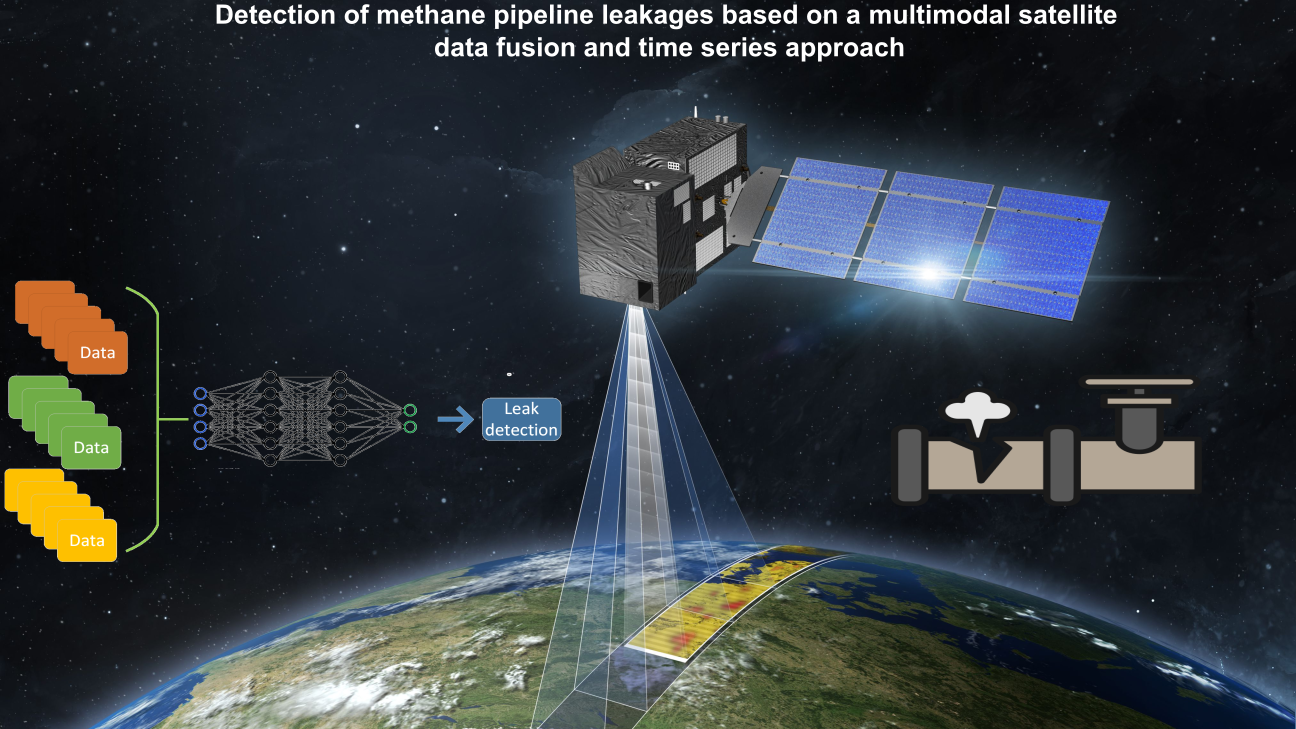