Cubesats have the potential to revolutionize Earth Observation by exploring different operating points in terms of spatial, temporal, and spectral resolution. However, physical limitations, mostly in terms of size, prevent them from obtaining high-quality images. OPS-SAT, while characterized by a small form factor, provides an impressive in terms of capabilities and flexibility hardware/software platform for executing demanding machine learning algorithms (inference stage). We propose to leverage these capabilities to address image quality limitations through the deployment of deep learning algorithms capable of substantially increasing the acquired image quality, in terms of PSNR, MSE, and relevant metrics, at a target GSD. This will be achieved by performing in-orbit multi-frame image super-resolution, effectively fussing short sequences of low-quality images into higher-quality ones. Effectively, the proposed approach employs computational imaging methods for increasing the spatial resolution (GSD) of the images, beyond the resolution imposed by the imaging hardware (optics, detector). In addition to providing higher spatial resolution images, the proposed approach will also offer significant advantages in terms of bandwidth needed for space-to-ground communications, by limiting the space-to-ground transmission to high-quality images only. The introduction of on-board image quality enhancement will also positively influence a wider range of applications, like object detection, which can also be deployed on-board.
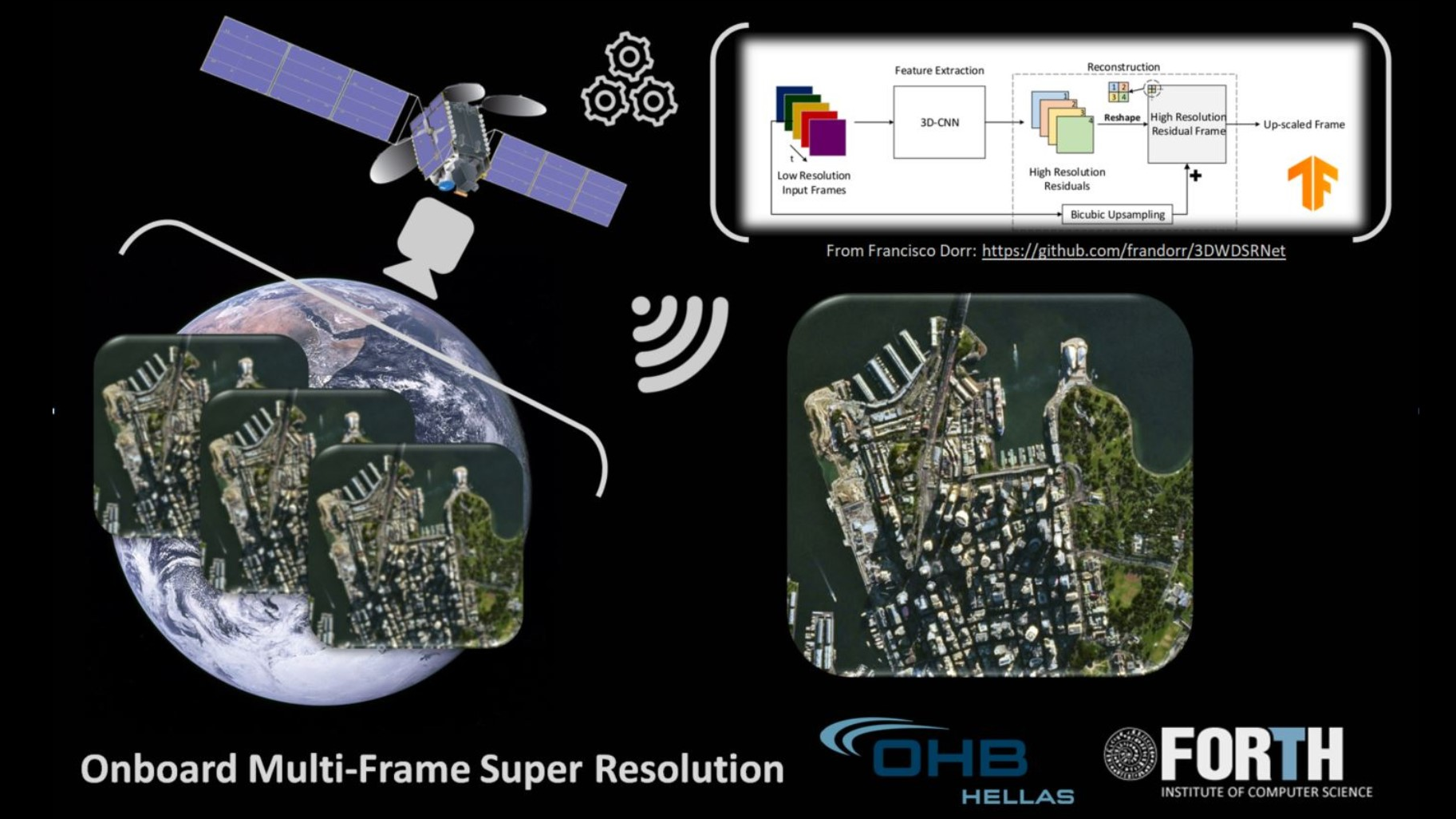