Duration: 5 months
The activity is framed within the PhD research project on visual relative navigation algorithms, development for the ESA mission SROC (Space Rider Observation CubeSat) in which close proximity operations and docking manoeuvres will be performed between a 12U CubeSat, as a chaser, and Space Rider as a target. Visual navigation for small satellites is a recent field and presents technological challenges. It is crucial for safety, especially during docking, as high precision is required. Moreover, the absence of real images of the target constraints the development of versatile and robust algorithms using synthetic images. Currently, the study focuses on developing a Convolutional Neural Network (CNN) based on state-of-the-art architectural advancements, using a multiple-heads architecture, and merging existing literature solutions with new features, consistent with the case study. However, a key challenge arises from the domain gap between the fully synthetic training dataset and real-world imagery. Data augmentation techniques are employed to mitigate this discrepancy. While unsupervised domain adaptation (UDA) holds promise, the proposed work aims at validating the developed algorithms through a hardware-in-the-loop (HIL) dataset. Alternatively, direct training on HIL images could further bridge the domain gap. Successful results from this study would demonstrate the feasibility of our CNN-based approach for CubeSat visual navigation and establish the importance of HIL validation for addressing the synthetic-to-real transfer challenge and will confer a higher confidence level on the effectiveness of the developed algorithms on board.
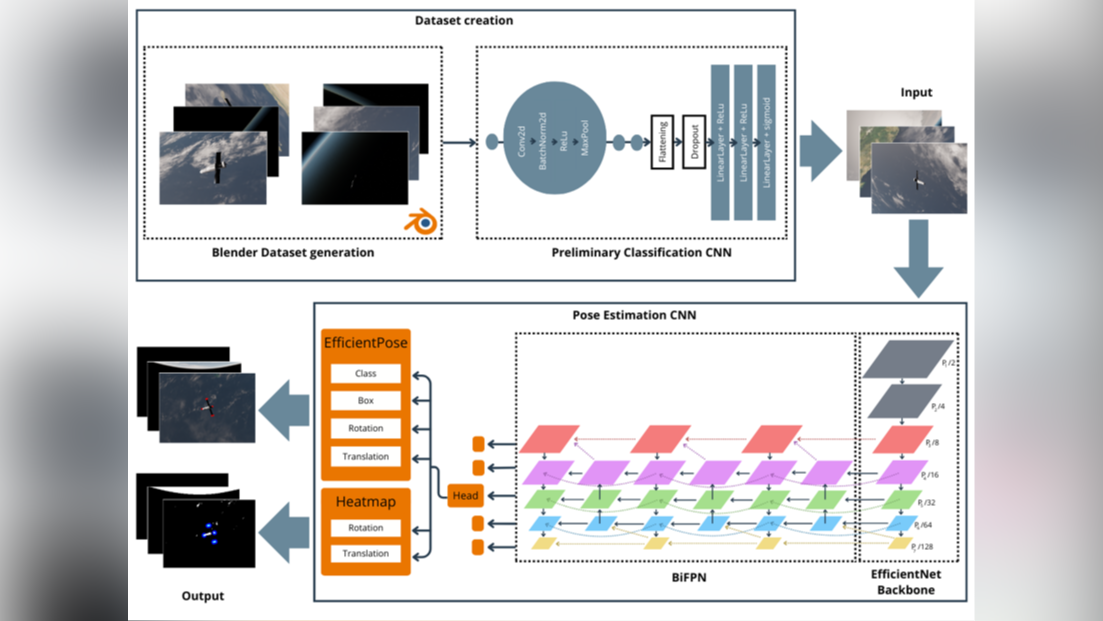