Duration: 36 months
In computer vision (CV) the development of transfer learning methods has enabled a significant progress of many specific object detection methods thanks to the use of accurate pre-trained image feature detectors trained on millions of images. To achieve a similar level of universalism in Earth Observations (EO) that has already been achieved in the field of CV, multi-scale EO databases are needed. Although there are many labelled EO databases, they do not have the key feature of computer vision databases that allows them to achieve universality, i.e. multi-scale (multi-sensor) class representation with wide spectrum of object categories. Models trained on multi-scale training data not only enable becoming independent from the problem of different data resolutions, but also allow to improve the overall detection accuracy at a given scale by focusing on identifying universal discriminatory features of a given object category.
This project responds to this gap in EO by the creation of the multi-scale global database based on a wide spectrum of optical satellite data and obiect categories in an amount and shape appropriate to fine-tune CV models. Thanks to this, models capable of detecting objects will be obtained, which are invariant with respect to image resolution. This will allow using the same models for EO images coming from different missions and open a path for more advanced multi-scale analyses. The creation of the mentioned database of labelled data will be done by combining data from existing EO datasets and selecting new examples to fill the gaps in these databases. Different pre-trained CNNs models will be fine-tuned on these data and their performance will be assessed. Practical use will be validated on the example of a real use case. The database and the Python implementation of the models will be publicly available to enhance further usage and research, following the success of similar approaches in CV domain.
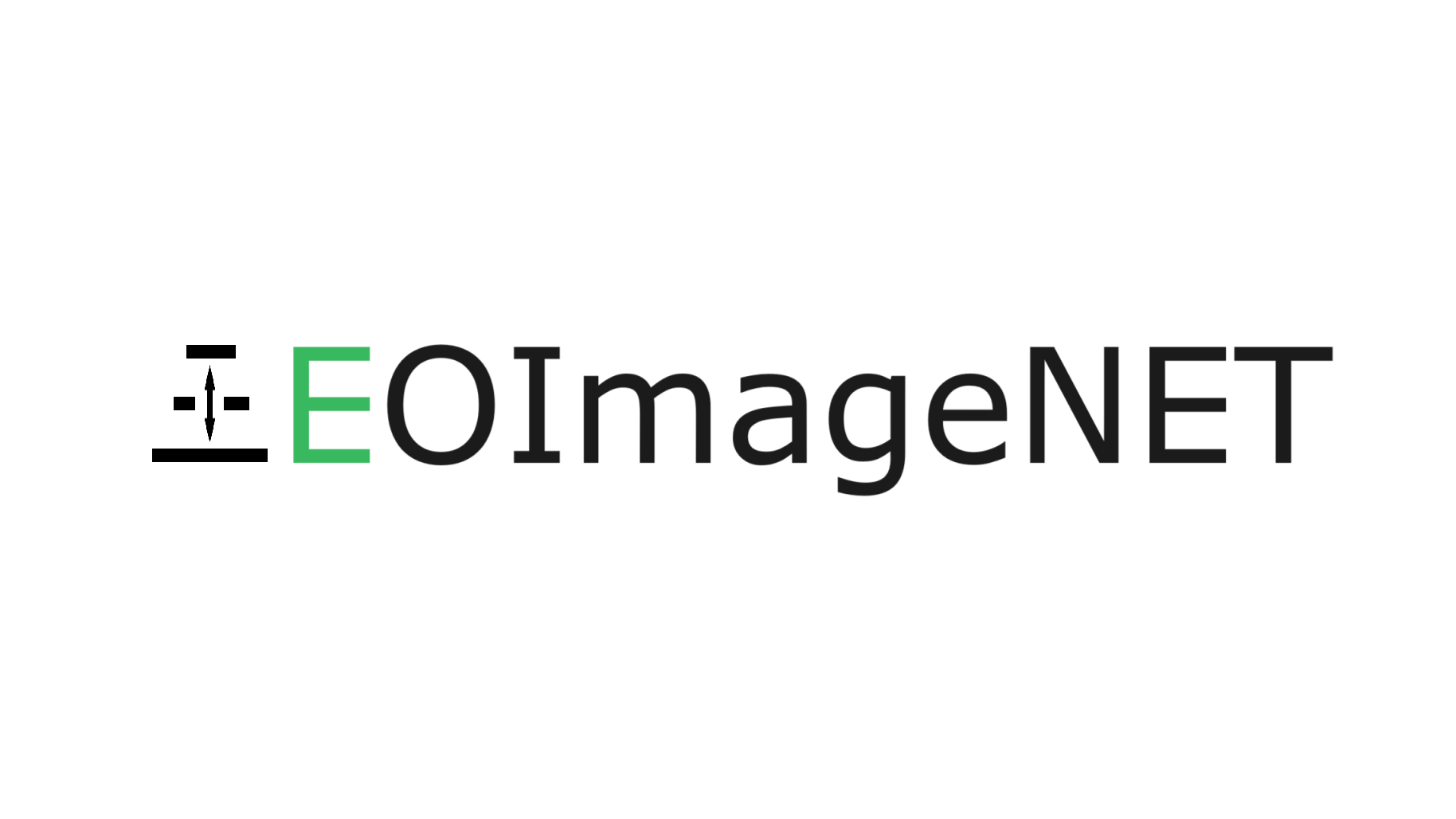