Duration: 18 months
Sea ice is essential to monitor for understanding the state of the climate, for weather prediction, and for sailing quickly and safely in the Arctic. For these applications, sea ice thickness is a crucial parameter. By measuring the freeboard relative to local sea ice level in sea ice leads, sea ice thickness can be estimated using satellite altimetry, such as CryoSat-2 (CS2) [1]. However, CS2 has sparse coverage, with an across-track footprint of 1600m, making it only adequate for monthly monitoring of sea ice thickness and insufficient for navigation. On the other hand, each Sentinel-1 (S1) [2] side-looking SAR image covers 400x400km in EW-mode, enabling extensive sea ice monitoring. S1, however, cannot directly map sea ice thickness, as the 5.55cm wavelength radar pulse is scattered by the surface, enabling only surface imaging. Sea ice thickness is indirectly mappable from S1, as professional sea ice analysts at national ice services produce so-called stage-of-development information, describing the age of the ice as a proxy for its thickness [3]. However, the sea ice thickness categories have considerable uncertainty and span, e.g. 30-200cm or 70-120cm [4]. Moving beyond using sea ice charts as label data towards physics-determined CS2 sea ice thickness derived from freeboard information [1] to train models would allow human-independent labels. This would increase the quality and availability of training labels with unbiased data and coverage extended beyond national boundaries otherwise mapped by national ice services. To improve sea ice thickness mapping, we propose to study the feasibility of training supervised deep-learning models to map CS2 sea ice radar freeboard from input S1 SAR images. A successful model could produce sea ice radar freeboard across the SAR image that is convertible to sea ice thickness, providing broader sea ice thickness mapping at higher revisiting frequencies, benefitting maritime navigation and climate and weather modelling.
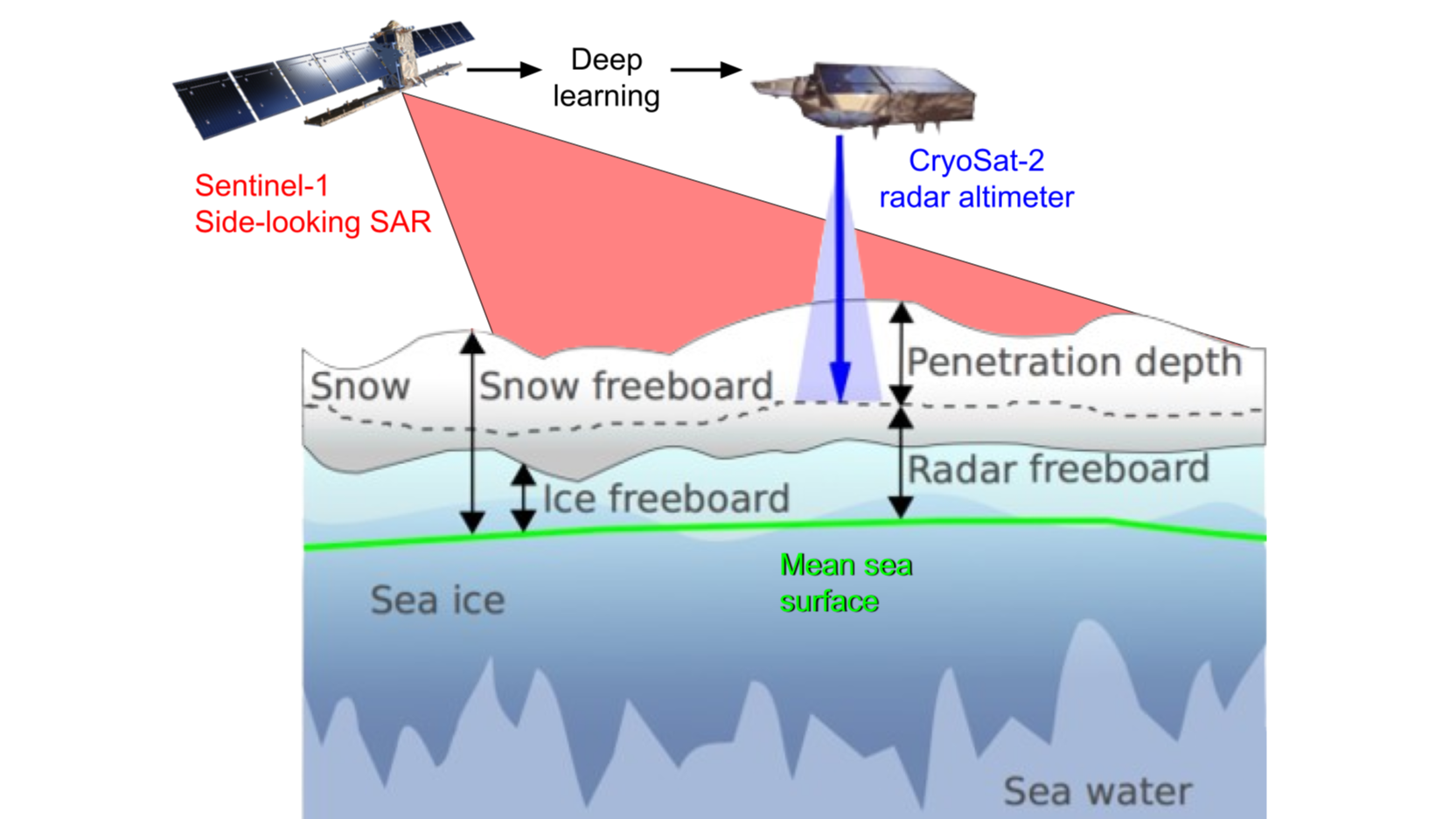