This research proposal is aiming at bringing together the latest research in explainable AI and probabilistic planning to equip future on-board autonomous spacecrafts with robust and interpretable mission planning and scheduling systems. Space systems capable of autonomously creating plans from sensing information, or re-planning in response to failures or unexpected events, would greatly improve the scientific return of a mission as well as increase system safety.
AI techniques has been largely applied to the design of autonomous systems that are able to cope with various level of uncertainties in the system, surrounding environment or mission goals. However, AI models generally lack of human interpretability. Addressing the "explainability” problem can provide insight on the autonomous planning and scheduling process, and its environment, by answering questions such as: how should a planning system explain particular ordering decisions, or resource choices? For humans, to be able to trust an AI system, they have to understand the underlying AI reasoning process in a way that is transparent and comprehensible.
Addressing the “robustness” problem can produce task plans and schedules that are robust to uncertainties. Incorporating probabilistic reasoning into the planning and scheduling system would lead to the generation of dynamically controllable strategies. This is achieved by including an intermediate layer between the planning and scheduling algorithm and the mission controller, called Abstract Argumentation (AA). AA will be able to justify robust solutions taken autonomously by the system both in terms of robustness as well as in terms of optimality in natural language. Two case studies will be simulated and analysed: fault recovery plan and observation plan for natural disaster monitoring.
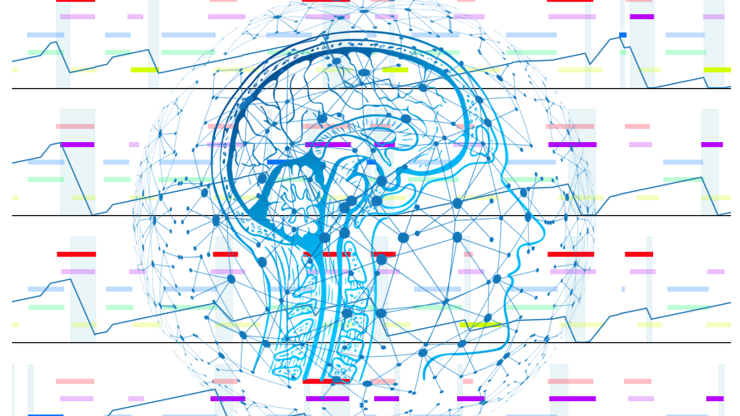