Remote detection and mapping of floating marine plastic litter (FMPL) from space needs to operate in synergy with clean up actions. This implicates that an observation system is needed combining high revisit and high spatial resolution. Currently there is no single remote sensing technology with sufficient revisit capabilities and spatial/spectral resolution relevant to FMPL dynamics. Therefore a combination of small drones, satellites and High Altitude Pseudo-Satellite (HAPS) is anticipated to provide the long term sustainable technical solution. The small drones are essential for calibration and validation of the HAPS and satellite datasets. We propose the use of small drones and in-situ observations combined with Artificial intelligence (AI) for this purpose. Machine learning has been shown to be useful in automated detection of beach litter (Martin et al., 2018). We want to go a step further by applying a similar AI-drone approach for the automatic detection of floating as well as slightly submerged marine plastic litter.
Our idea is to evaluate the performance of AI techniques for marine litter mapping using high spatial resolution true colour RGB or multi-spectral UAS imagery. The proposed AI-drone approach does not necessary imply the need for an hyperspectral SWIR sensor which is frequently proposed in literature as potential technique for marine litter detection, although hyperspectral SWIR data can be included in the AI analyses. RGB or multi-spectral drone sensors (compact and lightweight by design) are affordable hence easy to integrate on widely used drones compared to hyperspectral SWIR sensors (heavy due to design) requiring dedicated drones with large payload capabilities. Widely used drones by researchers, environmental managers and citizens offer a lot of potential validation opportunities. Results from the AI-drone approach will be compared with satellite imagery (e.g. Sentinel, WorldView, Pléiades, Planet) and evaluated as a Cal/Val tool.
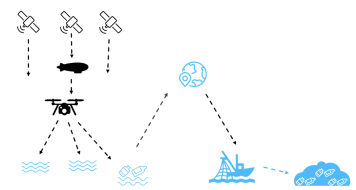