Remote sensing of plastic marine litter has been focused on finding spectral absorption patterns of plastic from high definition airborne imagery: Optical&SAR. number of research papers and symposiums[1],[2]. Remote sensing of plastic marine litter has been focused on finding spectral absorption patterns of plastic from high definition airborne imagery: Optical&SAR. These campaigns are indeed important for any validation and feasibility assessment of sustainable models of remote sensing. Indeed, S2 provides not only a worldwide coverage, but also the possibility to carry out temporal measurements of the detection area. An automatic detection model, will be able to be fed into dynamical transport models of plastic litter with marine current convection models predicting plastic litter transport dynamics. Detection model should be accurate enough to detect plastic in marine surface, coastal & rivers and to track the root of contamination. Thus, we could have a near real time of contamination sources facilitating priority actions & dissemination actions through social community actors[3]. We propose a novel methodology to detect plastic debris through deep Convolutional Neural Networks and ML to classify different type of spot debris. Deep CNN are very powerful methods to detect objects from images, being successfully applied for detection and segmentation of single pixels in multi band optical satellite data.[4]. Through learning process, in which segmentation output is compared to ground truth image, deep CNN adaptatively searches for optimal weights reducing error between the output of model and aground truth. Since the number of free parameters is generally very large, the CNN can learn several patterns that most physical models cannot. This 'supervised' learning model needs input of a ground truth mask. It could be produced by matching previous research on airborne images (S2 data). The output of this research is an automatic detection of plastic debris in S2 images though time.
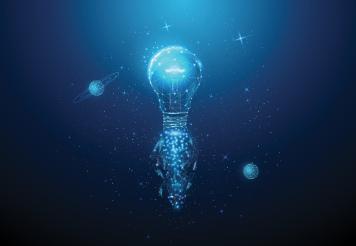