The objective of the activity will be to study data reduction impact from the usage of "local" optimum SAR data compression, adapting it to the type of scenario observed by the radar instrument. For "local" we refer to the characteristics in terms of backscattering and topographic heterogeneity of the scene observed. These characteristic lead to different dynamics and features of the received signal and therefore may be intercepted by Machine Learning trained algorithms, and will allow processing, tagging and selection directly on RAW data, thus selecting the best SAR data compression scheme depending on predefined application and frequency bandwidth. We expect this application to bring 10-30% overall science data reduction without impacting the data quality and entropy.
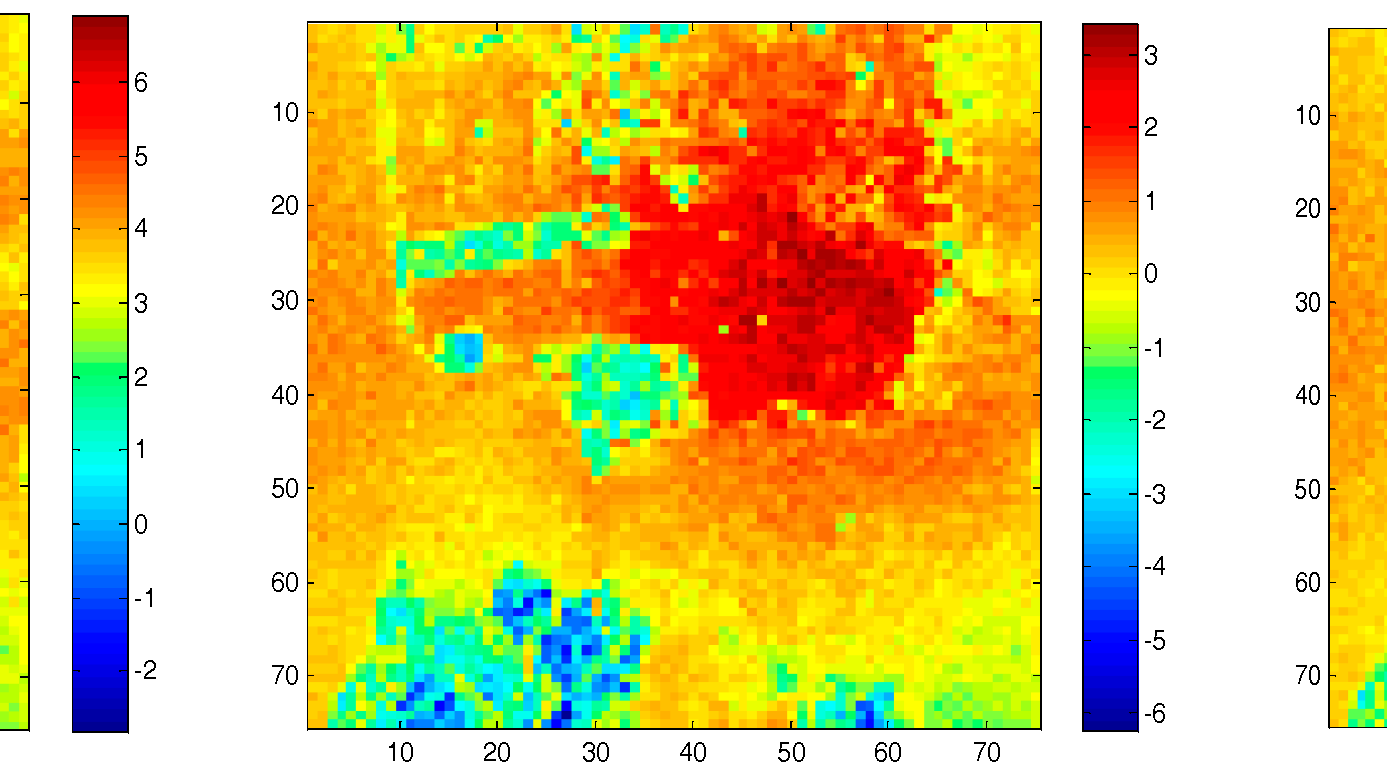