Duration: 38 months
Modern spacecrafts are becoming increasingly complex in order to satisfy the tighter performance requirements of upcoming missions, e.g., disturbance rejection, thermal and pointing performance requirements. Due to the complexity of their high-fidelity simulation models, the Verification and Validation (V&V) of Guidance, Navigation and Control (GNC) algorithms is costly and time-consuming. Model-based tools from robust control are highly effective to (in)validate GNC algorithms, to understand the impact of the modeled uncertainties on the performance signals, and to efficiently identify problematic worst-case parameter combinations for large uncertain models. These tools can be used as a preliminary analysis before starting the V&V campaigns, largely mitigating the need for re-tuning GNC solutions and re-starting the V&V campaigns. Building suitable models for this preliminary analysis is a challenging process, currently accomplished using linear approximations, which are restricted to specific use-cases, and with crude models to describe more complex features, such as the fuel sloshing. In this research, we aim at developing an automated surrogate modeling approach, which, from high-fidelity models that are pre-built in multi-body simulators, is capable to automatically extract simple surrogate models and learn the optimal trade-offs between model complexity, model conservatism and utilization objectives. Uncertainty of the original high-fidelity models and represented dynamics is also seamlessly incorporated in the surrogate models and refined with the help of measured mission data. The resulting automated surrogate modeling tool can have a dramatic effect on future missions by accelerating the model construction for the preliminary analysis and the entire V&V process of GNC solutions. Its efficiency will be demonstrated in high-fidelity simulators and experimental platforms.
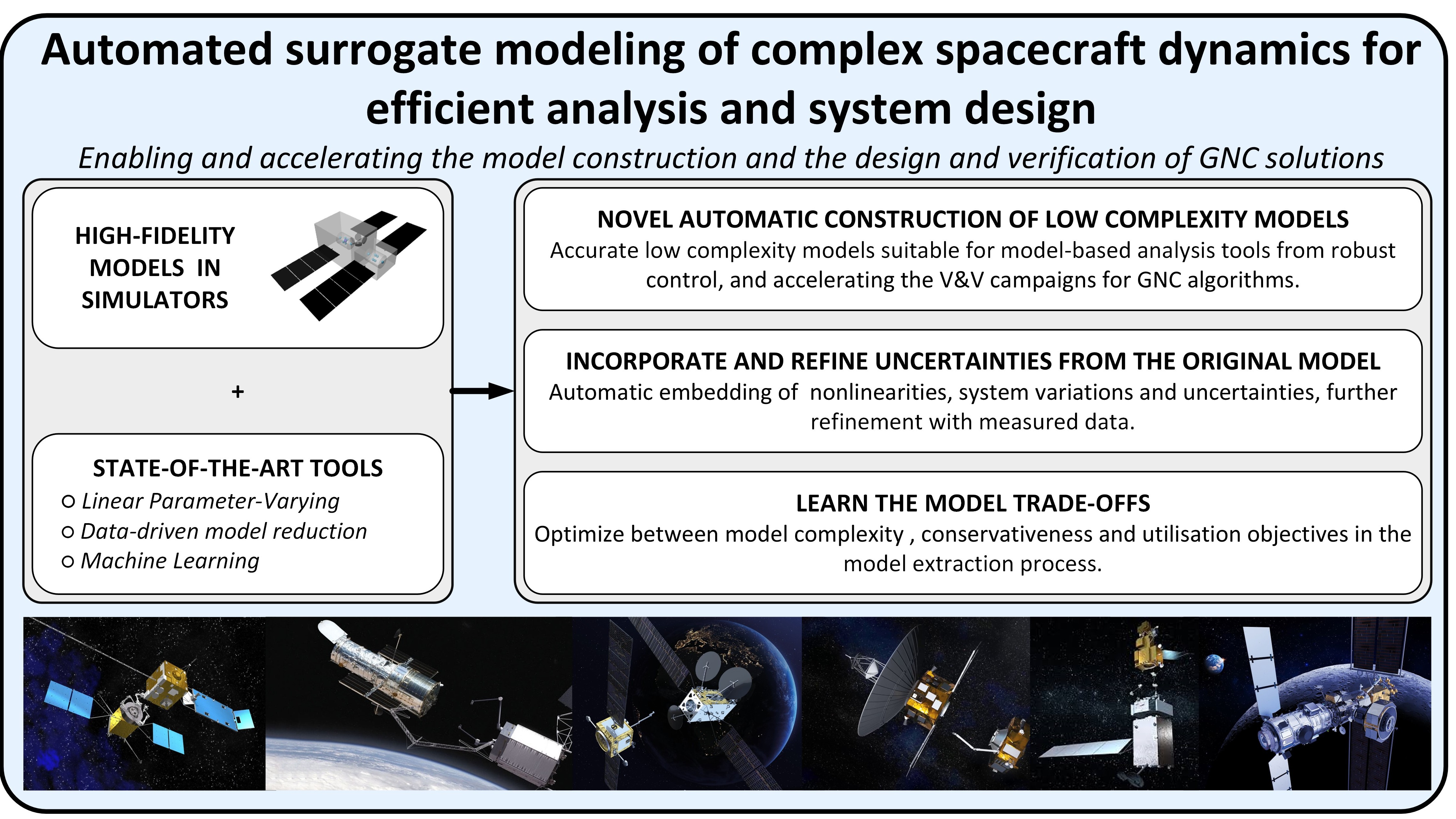